Rethinking Business Decisions: The Synergy of Decision Support System, Analytics, Business Intelligence, Artificial Intelligence, and Data Science
Amara Atif and M.A. Qureshi
Learning Objectives
By the end of this chapter, you will be able to:
LO1 Understand the history, development, and transformation of decision support systems over time.
LO2 Define decision support systems, explain its key features, and recognise its role in modern business environments impacted by technology, globalisation, and data growth.
LO3 Learn about the frameworks used to design decision support systems and understand how these frameworks guide decision-making processes.
LO4 Understand the five stages of the decision-making process and how decision support systems support each stage.
LO5 Identify the key characteristics, capabilities, and components of decision support systems, and examine how these elements work together to enhance decision-making.
LO6 Understand the key concepts and fundamentals of analytics, business intelligence, artificial intelligence, and data science, and explore the roles these disciplines play in supporting decision-making.
2 Transforming decision-making: The role of decision support systems in the modern business landscape
In today’s rapidly evolving business landscape, the ability to make informed and timely decisions can determine an organisation’s success or failure. As businesses face unprecedented challenges such as rapid technological advancements, globalisation, the digital economy, consumer behaviour shifts, and the explosion of data generation, these factors are redefining how businesses operate and compete. These changes are creating both challenges and opportunities, necessitating a transformation in how decisions are made. Traditional decision-making approaches, which often rely on intuition or historical data alone, are increasingly inadequate to address the speed, complexity, and scalability of modern challenges.
This chapter is essential to start with because it introduces the readers to Decision Support Systems (DSS) – a foundation for integrating data, technology, and analytics to enhance decision-making. It highlights how the evolution of DSS into modern technologies such as Analytics, Business Intelligence (BI), Artificial Intelligence (AI), and Data Science equips decision-makers with tools to navigate complexity, automate processes, and gain competitive advantages. Understanding these systems and their frameworks is key to unlocking better insights, making faster decisions, and driving innovation.
2.1 The evolution, definitions, and frameworks of decision support systems (DSS)
The development of DSS can be seen as a natural progression in the evolution of organisational information systems. This evolution began with Electronic Data Processing (EDP), which focused on automating routine, structured tasks, and progressed through Management Information Systems (MIS), which aimed to generate structured reports for managerial decision-making. The emergence of DSS represents the next step in this trajectory, addressing the need for systems capable of handling complex, unstructured problems.
Defining decision support systems
Decision support systems are characterised as interactive, computer-based systems designed to assist decision-makers in solving unstructured or semi-structured problems by utilising data and models.
Unlike EDP and MIS, which focus on predefined tasks and structured reports, DSS offers flexibility and interactivity, enabling users to explore data dynamically and simulate various scenarios to arrive at informed decisions.
Expanding perspectives on decision support systems
While traditionally defined as tools for aiding decision-making through data and models, some authors and researchers have broadened the scope of DSS to include any system that contributes to decision-making. This broader perspective implies that virtually all systems, except for basic transaction processing, could be considered a DSS. However, this overly inclusive definition risks diluting the concept, making it challenging to distinguish DSS from other systems or to understand their specific value and technical requirements.
Redefining decision support systems: The case of Navigation Apps
For example, a navigation app such as Google Maps illustrates the broader perspective on what could be considered a DSS. Such an app collects real-time data (traffic conditions, road closures), utilises models (shortest route algorithms, estimated arrival times), provides interactive options (alternative routes, live traffic updates), and assists users in making decisions (which route to take based on time, traffic, or preferences). From a broader viewpoint, this app “supports decisions” by helping users choose the best travel route, making it a potential DSS.
However, if the definition of DSS is applied too broadly, systems like navigation apps or even weather apps could fall under the DSS umbrella, even though they may lack the depth of functionality or technical requirements traditionally associated with DSS, such as advanced “what-if” analysis or scenario modelling.
Frameworks for understanding decision support systems
A framework is a structured and organised approach to developing or understanding a concept, system, or process, providing a blueprint or guidelines to systematically analyse, design, or implement solutions. It emphasises structure by defining interconnected components, offers guidance through principles and practices, and remains flexible and scalable to adapt to varying contexts or project sizes. Frameworks are widely used across disciplines, from technology to management, to simplify complex problems and ensure consistency in methodology.
In the context of DSS, frameworks play a critical role in organising thoughts and enhancing decision-making by breaking down tasks into manageable parts. Researchers often develop DSS frameworks tailored to their specific projects, ensuring that the systems address the unique requirements of the problems being studied. A good starting point for understanding DSS frameworks is the classic model developed by Gorry and Scott-Morton’s “A framework for management information systems” (1971), which uses a 3×3 matrix to categorise decisions based on problem structure (ranging from structured to unstructured) and organisational level (from operational to strategic). This foundational framework highlights the necessity of integrating data, models, and user interactivity to effectively support decision-making processes.
Challenges in defining decision support systems
The term decision support system holds intuitive appeal, as any system that aids a decision might seem to qualify. Yet, restrictive definitions fail to fully capture the diverse functionalities of DSS, while overly broad definitions lack practical utility. Additionally, people from varying professional backgrounds and organisational context’s view DSS differently, further complicating the development of a unified definition. This ambiguity highlights the importance of foundational works, such as Alter’s “A taxonomy of decision support systems” (1977), to gain clarity on the core principles and design approaches of DSS.
2.2 Changing nature of business environments and evolving needs for decision support and analytics
Modern businesses are experiencing significant changes driven by rapid technological advancements, global interconnectedness, and increasing volumes of data. To thrive in this fast-changing environment, businesses are turning to DSS powered by data-driven technologies. These tools help businesses make quicker and smarter decisions. Below are the key drivers of change in business environments, with examples from Australian businesses included to illustrate their impact.
2.2.1 Rapid technological advancements
Emerging technologies such as the AI, machine learning, Internet of Things (IoT), and cloud computing are transforming how businesses operate. These innovations enable the collection and analysis of vast amounts of data in real-time, providing valuable insights for decision-making.
Example
2.2.2 Globalisation
Globalisation has increased competition by allowing businesses to operate across borders, exposing them to diverse markets, regulations, and cultural nuances. This has amplified the need for agile decision-making tools.
Example
2.2.3 Massive data generation
The explosion of digital channels, IoT devices, and customer touchpoints generates massive amounts of structured and unstructured data. Managing and extracting actionable insights from this data is essential for businesses to remain competitive.
Example
2.2.4 Consumer expectations
Modern consumers demand personalised experiences, rapid service, and ethical business practices. Businesses that fail to meet these expectations risk losing customer loyalty.
Example
2.2.5 Uncertainty and volatility
Unpredictable events, such as the COVID-19 pandemic and geopolitical tensions, have introduced unprecedented levels of uncertainty and volatility. These disruptions highlight the importance of robust and flexible decision-making systems.
Example
2.3 The transformation of decision support systems over time
Over decades, DSS has transformed from static tools limited to structured data into sophisticated systems powered by AI and real-time processing. This evolution of DSS reflects the growing complexity of the business world and the increasing demand for agility, efficiency, and accuracy.
For Australian businesses, this journey highlights the importance of innovation in maintaining competitiveness. Whether it’s a small grocer relying on basic systems or a multinational retailer using AI to predict consumer behaviour, DSS continues to shape the way decisions are made.
Early DSS: The beginning of data-driven decisions
The story of DSS begins in the 1960s and 1970s when businesses started using basic systems to assist decision-making. These early DSS were simple, focusing on structured data stored in databases and generating static reports. For instance, a retailer might have used these systems to track inventory levels manually and generate monthly stock reports. While groundbreaking at the time, these systems lacked the ability to handle dynamic environments or real-time changes.
Example: A local Australian grocer using a basic system to calculate stock levels for staples such as bread and milk manually and generate monthly stock and sales reports.
Executive Information Systems (EIS): Putting insights in the hands of leaders
By the 1980s, the rise of EIS brought DSS into the boardrooms of senior executives. These systems provided high-level summaries and dashboards that allowed decision-makers to quickly grasp business performance. Unlike their predecessors, EIS featured customisable visualisations and drill-down capabilities, giving leaders a clearer picture of their operations.
Example: An Australian manufacturing company, such as BlueScope Steel or Boral, might use an EIS to monitor key metrics related to its production processes and financial health. For instance, if the company notices that a particular factory consistently has higher costs due to overtime caused by machine breakdowns, the EIS might recommend redirecting funds to upgrade or repair machinery. Executives can then adjust their strategy to reduce costs and increase efficiency, ultimately improving profitability.
Business Intelligence (BI): Interactive insights for better decisions
In the 1990s, BI tools revolutionised DSS by introducing interactive capabilities. BI allowed businesses to integrate data from multiple sources and generate detailed analyses. For the first time, decision-makers could go beyond static reports and explore data dynamically through ad-hoc querying and visualisations.
Example: A major Australian retailer such as Myer or David Jones using BI to analyse seasonal sales trends across multiple store locations, identifying which regions required restocking or promotional efforts.
Analytics and big data: Harnessing the power of vast data sources
The early 2000s marked the era of analytics and big data, where DSS grew smarter and more powerful. Businesses started having access to advanced statistical models and machine learning methods to process vast amounts of both structured and unstructured data. This shift enabled predictive and prescriptive analytics, allowing organisations to anticipate trends and make proactive decisions.
Example: Australian logistics companies such as Toll Group using predictive analytics to optimise delivery routes by factoring in real-time traffic, weather conditions, and customer locations.
Automation and AI: The rise of intelligent decision systems
The integration of automation and AI into DSS represents the latest stage in their evolution. These advanced systems have the capabilities to analyse vast amounts of data in real-time, dynamically adapt to changing conditions, and even autonomously execute decisions. By automating routine and repetitive tasks, these intelligent systems allow human resources to focus on more strategic and creative activities, ultimately improving efficiency and decision-making speed.
Example: Woolworths leverages AI-driven DSS to manage inventory in real-time, ensuring shelves are stocked with high-demand items during peak periods, such as holiday seasons or unexpected surges. For instance, during the COVID-19 pandemic, the system anticipated spikes in demand for essential items like toilet paper and hand sanitiser, enabling proactive restocking to meet customer needs.
2.4 Decision-making process
Decision-making is a structured and systematic process that enables organisations to address challenges or seize opportunities effectively. This process comprises five key stages, each critical to achieving informed and successful outcomes. Figure 2.1 provides a visual representation of these stages in the decision-making process.
Stage 1: Intelligence
The first stage involves identifying and defining the problem or recognising a potential opportunity. DSS supports this stage by providing tools to collect and consolidate data from various sources, such as market trends, operational metrics, and customer feedback, into a unified platform. Advanced analytics within DSS can detect patterns, identify anomalies, and flag potential opportunities automatically.
Stage 2: Design
In this stage, potential solutions are developed by brainstorming and exploring different options. DSS helps by providing tools to simulate scenarios and predict outcomes of various strategies. This allows decision-makers to test how feasible each solution is and understand the risks and impacts.
Stage 3: Choice
After identifying alternatives, the next step is to evaluate their strengths and weaknesses and choose the best option based on factors such as cost, risk, and potential benefits. DSS supports this process by providing tools such as decision matrices[1], optimisation algorithms[2], and visualisations. These tools simplify complex comparisons and make the decision-making process more objective and efficient.
Stage 4: Implementation
This stage focuses on putting the chosen solution into action. For implementation to succeed, teams must work together effectively, progress needs to be monitored closely, and objectives must be met as planned. DSS supports this process by providing dashboards and real-time monitoring tools to track progress. It also offers alerts and recommendations if the project deviates from the plan, helping to address issues quickly and keep the implementation on track.
Stage 5: Evaluation
The final stage assesses how well the decision, and its implementation worked. This includes comparing actual results to the original goals, identifying successes, and finding areas for improvement. Lessons from this stage help refine future decisions. DSS supports this process by creating performance reports, highlighting what went well, and pointing out where results fell short. The system also uses feedback from these assessments to improve future decision-making strategies, enabling continuous improvement.
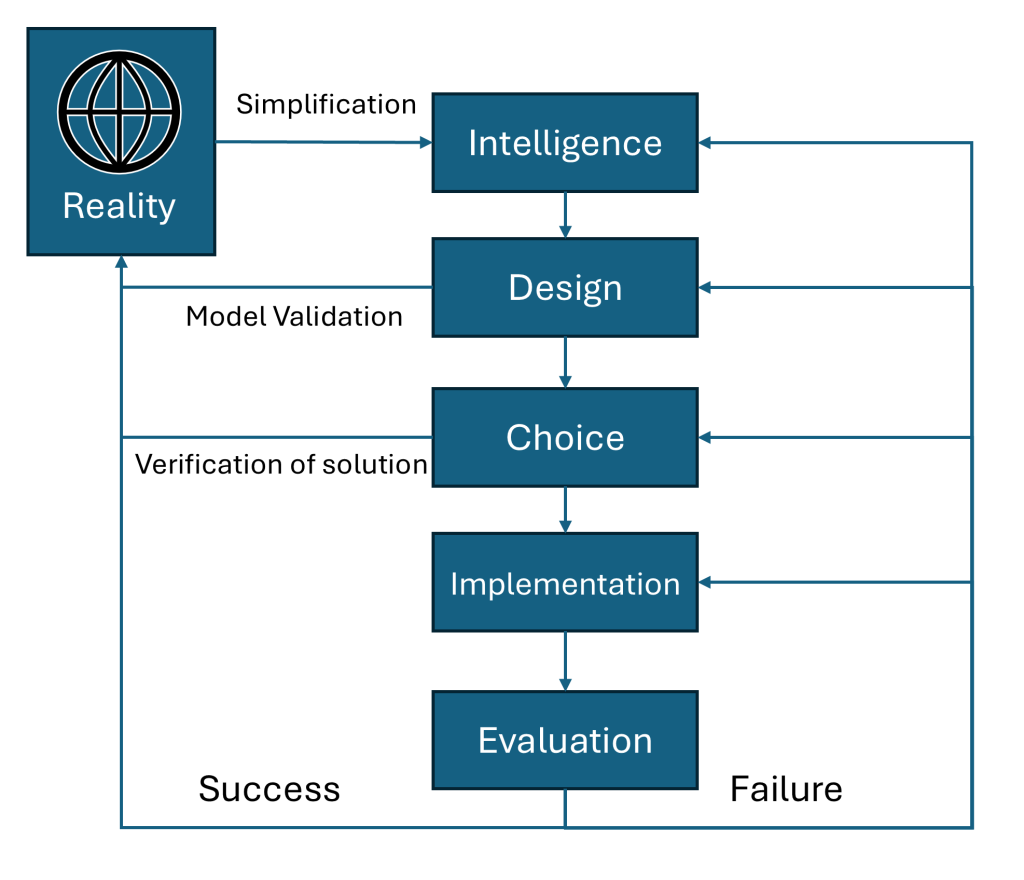
Alternative Text for Figure 2.1
A flowchart of the decision-making process, illustrating five stages: Intelligence, Design, Choice, Implementation, and Evaluation, with feedback loops for validation and verification leading to success or failure.
A common misconception
The decision-making process is the same as the BI development life cycle. The answer is No – they are distinct concepts, although they can overlap in the context of decision support.
The decision-making process refers to the steps individuals or organisations take to identify problems, evaluate options, and choose the best solution. On the other hand, the BI development life cycle focuses on creating and deploying a BI system – a technical and procedural approach to building the infrastructure needed for data analysis and reporting.
While the BI development life cycle supports decision-making by providing the tools and systems needed for data-driven decisions, they serve different purposes. The BI life cycle will be discussed in detail in Chapter 9.
Example business scenario: Implementing a new product line in an Australian retail chain
An Australian retail chain, AussieMart, is considering introducing a new line of eco-friendly household cleaning products. This scenario describes how the decision-making process and its five stages help the company address this opportunity effectively.
Stage 1: Intelligence
The management team identifies a growing demand for eco-friendly products among Australian consumers, driven by sustainability trends and environmental awareness. DSS supports this stage by integrating and analysing data from multiple sources:
- Market trends: DSS tools consolidate external reports, highlighting a 25% year-on-year growth in the eco-friendly product segment.
- Operational metrics: DSS dashboards analyse historical sales data and detect declining demand for non-eco-friendly products.
- Customer feedback: Sentiment analysis through DSS tools reveals that 70% of AussieMart’s customers are willing to pay more for sustainable options.
Through these capabilities, the DSS not only helps gather data but also provides actionable insights, such as pinpointing customer preferences and emerging market trends, which allow AussieMart to clearly define the opportunity to launch a new product line. This ensures the organisation is well-prepared to move into the design stage.
Stage 2: Design
The team explores different strategies for launching the product line such as partnering with a local supplier for a co-branded product, importing products from a well-known international brand, and developing an in-house eco-friendly brand.
Using their DSS, they simulate scenarios for each option:
- Scenario modelling: Predicting potential costs, revenue, and market share for each strategy.
- Risk analysis: Evaluating risks like supply chain disruptions, initial investment, and brand alignment.
This stage helps the team understand the feasibility, risks, and impacts of each option, ensuring they are well-prepared to evaluate alternatives.
Stage 3: Choice
The team uses DSS tools to objectively compare the three alternatives:
- Decision matrices: A table evaluates each option based on key criteria such as cost, customer appeal, and time to market. Scores indicate that partnering with a local supplier is the most balanced choice.
- Optimisation algorithms: These helps identify the optimal pricing strategy for maximising revenue while staying competitive.
- Visualisations: Charts and heatmaps show the projected profitability of each option over three years.
Based on the insights, AussieMart decides to partner with a trusted local supplier to co-brand an eco-friendly cleaning product line.
Stage 4: Implementation
To launch the new product line, the team coordinates across departments:
- Procurement works with the local supplier to finalise product specifications.
- Marketing designs a campaign emphasising sustainability and local partnership.
- Operations ensures distribution to stores and online channels.
The DSS provides:
- Dashboards to track the production, distribution, and marketing activities in real-time.
- Alerts to notify the team of delays in packaging or supply chain issues.
- Recommendations suggesting adjustments to distribution if certain regions show higher demand.
The coordinated efforts ensure that the product is launched on schedule and meets customer expectations.
Stage 5: Evaluation
After the product launch, the team evaluates its performance:
- Sales metrics: Reports indicate a 20% increase in sales in the household cleaning category.
- Customer feedback: Positive reviews highlight the product’s eco-friendly benefits and affordability.
- Profitability analysis: The DSS compares actual revenue with projections, showing the product exceeded expectations by 10%.
The evaluation identifies areas for improvement, such as optimising supply chain logistics to reduce costs further. Lessons from this launch are incorporated into future product strategies, ensuring continuous improvement.
By following the structured decision-making process and utilising DSS at every stage, AussieMart successfully capitalises on a market opportunity. DSS tools play an integral role in supporting all stages – from identifying the opportunity in the intelligence stage to evaluating the success of the product launch in the evaluation stage. This approach ensures data-driven decisions, continuous learning, and long-term growth.
2.5 Decision support system characteristics, capabilities, and components
2.5.1 Characteristics and capabilities of decision support systems
The diverse nature of DSS stems from the lack of consensus on its exact definition, leading to varying interpretations of its standard characteristics and capabilities. To better understand this diversity, the key characteristics and capabilities of DSS can be grouped into four broad categories. As depicted in Figure 2.2, these categories encompass essential functionality, user support, process enhancement, data and tools, and technical versatility. The characteristics and capabilities highlighted are drawn from the works of Alter (1977), Sprague (1980), Sharda (2018), and others, and include:
- Core functionality and adaptability: DSS is designed to handle not just structured problems but also semi-structured and unstructured problems, making it highly versatile. Its flexible nature allows it to adapt to a wide range of decision-making contexts, providing effective solutions for diverse challenges. This makes DSS equally valuable for structured tasks, like inventory management, and more ambiguous, unstructured challenges, such as long-term strategic planning or market entry analysis.
- User support and interactivity: DSS provides comprehensive support to individuals, teams, and managers, emphasising ease of use and adaptability to user needs. Its interactive interfaces allow users to manipulate data, test scenarios, and explore outcomes in real-time, fostering better understanding and collaboration.
- Process enhancement and data integration: DSS enhances decision-making effectiveness by supporting all stages of the decision process, from problem identification to evaluation. Its data-driven approach integrates structured (e.g., sales data) and unstructured data (e.g., customer feedback), empowering users with deep insights.
- Analytical tools and technical versatility: DSS offers advanced analytical tools and models, including decision matrices, optimisation algorithms, and visualisations, to simplify complex comparisons. Its technical versatility ensures it can function as a standalone system, integrate with existing platforms, or operate as a web-based solution.
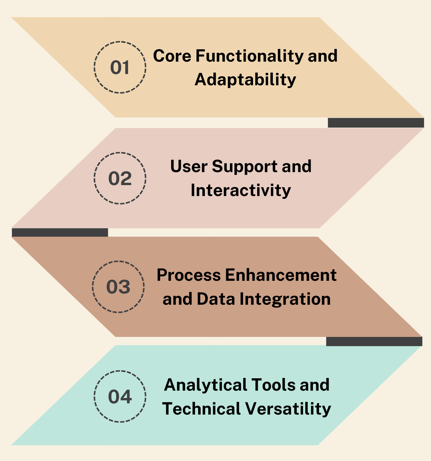
Alternative Text for Figure 2.2
A diagram representing the four primary characteristics of Decision Support Systems:
1) Core Functionality and Adaptability,
2) User Support and Interactivity,
3) Process Enhancement and Data Integration, and
4) Analytical Tools and Textual Versatility, displayed in a stepwise format.
Business scenario: DSS in supply chain management for an Australian retail industry
To illustrate the characteristics and capabilities of DSS, consider the scenario of a large Australian retail company, such as a supermarket chain managing its supply chain operations. Retailers in Australia often face semi-structured and unstructured problems, such as predicting demand fluctuations during public holidays (e.g., Australia Day), optimising inventory across remote or regional stores, and coordinating with local and international suppliers. Table 2.1 below outlines how a DSS designed for supply chain management demonstrates its characteristics and capabilities (Column 1), applies to real-world scenarios (Column 2), and serves specific applications (Column 3):
Characteristic/Capability |
Scenario |
Application |
Core functionality and adaptability |
The company uses DSS to address the semi-structured problem of restocking inventory during peak seasons. The system predicts demand using historical sales data, current trends, and external factors like holidays or economic shifts. |
DSS supports diverse decision-making styles, from analytical (data-driven forecasting) to heuristic approaches (experience-based decisions). It enables interdependent decision-making by integrating with logistics planning, ensuring seamless coordination between inventory levels and supply chain operations. |
User support and interactivity |
Managers at all organisational levels from warehouse operators to supply chain executives use DSS to coordinate inventory and supplier activities effectively. |
The DSS provides a user-friendly interface that allows non-technical users to generate reports, adjust restocking parameters, and analyse outcomes. It supports team collaboration by enabling multiple users to work simultaneously, share insights, and make collective decisions in real-time. |
Process enhancement and data integration |
During unexpected disruptions, such as a delayed shipment, the DSS helps the supply chain team analyse alternative suppliers or rerouting options in real-time. |
The application of DSS supports all stages of the decision-making process in a seamless and integrated manner. During the intelligence stage, the system identifies disruptions and assesses their impact on operations. In the design stage, DSS helps explore alternative solutions, such as evaluating different suppliers or rerouting options. Moving to the choice stage, the system evaluates these alternatives by analysing costs, timelines, and reliability to determine the most suitable option. Finally, in the implementation stage, DSS facilitates the execution of the selected solution, ensuring that decisions are carried out efficiently and effectively. This comprehensive approach allows the team to manage tight deadlines while maintaining full control of the decision-making process. |
Analytical tools and technical versatility |
The company integrates DSS with its sales and supplier database to provide comprehensive insights into historical sales, supplier reliability, and transportation costs. |
The DSS leverages advanced analytical tools like predictive models to generate actionable insights, such as optimal inventory levels or prioritising reliable suppliers. Its technical versatility ensures it can function as part of a larger Enterprise Resource Planning (ERP) system while providing web-based access for regional managers, allowing flexibility for both centralised and remote operations. |
2.5.2 Components of decision support systems
Figure 2.3 illustrates the key components of a DSS, which integrates various elements such as data, models, knowledge, and interactivity to support managers in making informed decisions. Below is a brief description of these components and their essential roles in facilitating effective decision-making.
01 Data management
Data management serves as the foundation of the DSS, responsible for collecting, storing, and organising data from various sources. These sources include internal systems, such as operational data from ERP or Point of Sale (POS) systems, legacy systems (older databases or applications still in use), and external sources, such as web services, [3] (APIs), or public databases.
02 Model management
Model management provides the analytical tools necessary for decision-making. It includes statistical models (e.g., regression analysis, time series analysis, clustering), optimisation techniques, and simulation tools (e.g., spreadsheet simulations, Monte Carlo simulation) that allow users to explore different scenarios and conduct “what-if” analyses.
03 External models
External models enhance the system by integrating specialised software, industry-specific algorithms, or AI models that might reside outside the core DSS. This enables access to industry-specific expertise or advanced computational capabilities.
04 Knowledge-based subsystems
Knowledge-based subsystems add another layer of intelligence to the DSS by leveraging organisational knowledge and domain expertise. This includes best practices, rules, heuristics, and accumulated expertise, often supported by AI or expert systems. These subsystems ensure that decisions are contextually informed and aligned with organisational goals.
05 User interface for end-user/decisionmaker
The user interface acts as the bridge between the system and the end-user or decision-maker (e.g., manager, business analyst). It offers an interactive platform, such as dashboards, where users can input preferences or assumptions, explore insights, and visualise reports, trends, and analysis results. It ensures the system remains user-friendly, facilitating intuitive interaction and delivering actionable insights in an accessible and comprehensible format.
06 Organisational knowledge base
The organisational knowledge base supports the entire system by storing critical information such as historical data, policies, and guidelines. This provides essential context for decision-making.
07 Interaction with external systems
The DSS interacts seamlessly with other computer-based systems, such as databases, customer relationship management (CRM) systems, or supply chain systems. It also connects to external networks, such as the internet or intranets, to gather real-time data, including global market data or competitor analysis. Together, these interactions ensure a comprehensive and effective decision-making process.
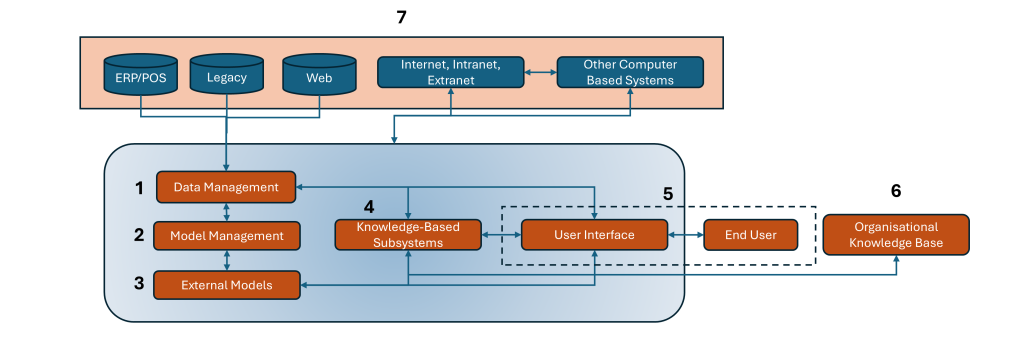
Alternative Text for Figure 2.3
A detailed architecture diagram of a Decision Support System (DSS), featuring interconnected components: Data Management, Model Management, External Models, Knowledge-Based Subsystems, User Interface, End User, and Organisational Knowledge Base. These components interact with external systems such as ERP/POS, legacy systems, and web-based solutions.
Example: A Bushfire management DSS
Australia frequently faces bushfires and managing them effectively requires data-driven decisions. A Bushfire Management DSS used by emergency services such as the NSW Rural Fire Service integrates various components to help decision-makers assess risks, allocate resources, and protect communities.
How it works in practice?
During a bushfire, the system gathers weather and satellite data, runs predictive fire spread models, and integrates knowledge from past bushfires. Emergency managers interact with the DSS through an intuitive dashboard, visualise the predicted fire behaviour, and make critical decisions, such as allocating firefighting resources and planning evacuations.
Components of the Bushfire Management DSS
Components of the Bushfire Management DSS are critical in enabling effective decision-making during emergencies by integrating real-time data, predictive models, and user-friendly interfaces to assist emergency managers. Table 2.2 includes the components and practical applications of a Bushfire Management Decision Support System.
Component |
Source |
Function |
Example in use |
Data management |
Real-time data from satellites, weather stations, drones, and ground sensors |
Collects and stores data on temperature, wind speed, humidity, and vegetation dryness |
Data from the Bureau of Meteorology (BoM) is integrated into the system to predict fire spread |
Model management |
Simulation and prediction models |
Uses fire spread algorithms and weather forecast models to simulate bushfire behaviour |
A fire simulation model predicts how a fire will spread based on wind patterns and vegetation |
External models |
Advanced fire prediction models or climate models developed by external institutions such as CSIRO (Australia’s national science agency) |
Provides detailed forecasts and long-term fire risk assessments |
A climate model predicts areas of high fire risk for the upcoming season |
Knowledge-based subsystems |
Historical data on bushfire patterns, expert knowledge from firefighters, and lessons learned from past incidents |
Adds context to the models by incorporating expert advice, past fire management strategies, and rules for resource allocation |
Knowledge from previous large bushfires helps prioritise which areas to evacuate first |
User interface for end-user/decision-maker |
Dashboards and visualisations accessible by fire management officers and emergency coordinators |
Provides an interactive platform for users to input data, explore scenarios, and visualise outputs |
Emergency managers use a dashboard to evaluate predicted fire paths, resource needs, and evacuation timelines |
Organisational knowledge base |
Policies, emergency protocols, and historical incident records stored within the system |
Supplies additional context and supports compliance with national fire management guidelines |
Accessing past incident records to refine evacuation plans and strategies |
Interaction with external systems |
Integration with external agencies like the BoM, local councils, and fire services |
Ensures collaboration and access to external data for comprehensive decision-making |
Synchronisation with local government systems to issue evacuation orders and community alerts in real-time |
2.6 Analytics vs Business Intelligence vs Artificial Intelligence vs Data Science
2.6.1 Overview and historical context
2.6.1.1 Analytics
According to the Institute for Operations Research and the Management Sciences (INFORMS), analytics is defined as the scientific process of transforming data into insight for making better decisions.
This definition emphasises the systematic approach of utilising data analysis to derive actionable insights that enhance decision-making processes. Analytics has its roots in statistics and mathematics, which date back centuries. Formal methods such as regression analysis began emerging in the late 1800s. By the mid-1900s, analytics evolved further with the introduction of computational tools and statistical software. It is the foundational concept that laid the groundwork for more specialised fields. Figure 2.4 illustrates the categories of business analytics, highlighting its evolution and applications in modern decision-making.
INFORMS further categorises analytics into three distinct types:
- Descriptive (or reporting) analytics: Provides insight into past events by analysing historical data.
- Predictive analytics: Offers foresight into future events by identifying patterns and trends.
- Prescriptive analytics: Recommends actionable strategies to achieve desired outcomes.
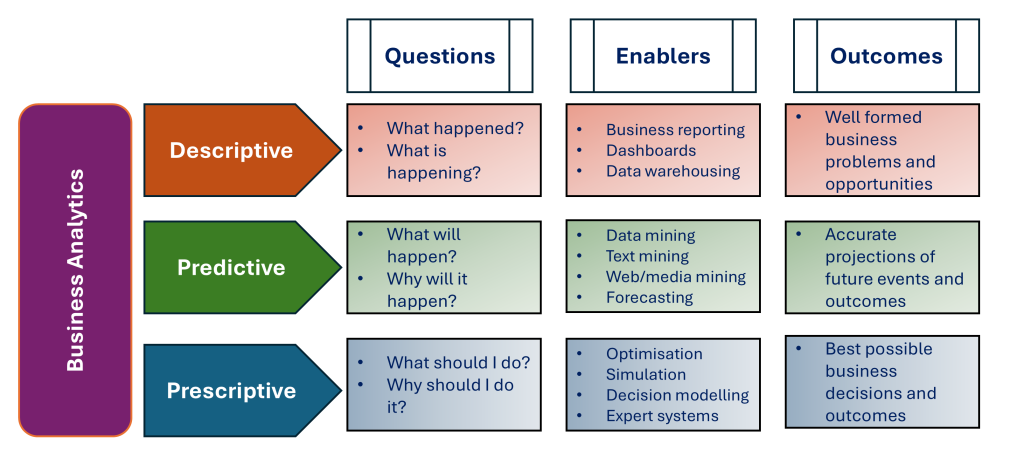
Alternative Text for Figure 2.4
A layered framework of business analytics types: Descriptive, Predictive, and Prescriptive analytics. Each layer highlights its associated questions (e.g., What happened?), enabling tools (e.g., dashboards, data mining), and outcomes (e.g., actionable insights or decision optimisation).
2.6.1.2 Business Intelligence
Business Intelligence (BI) refers to the technologies, processes, and practices used to collect, analyse, and present business data to help organisations make informed decisions. It involves gathering data from various sources, transforming it into actionable insights, and presenting it through visualisations, reports, and dashboards.
Key elements of BI include data mining, querying, reporting, and online analytical processing (OLAP), which help to improve efficiency, optimise processes, and support decision-making at all levels of an organisation. The term “business intelligence” was first used by Richard Millar Devens in his 1865 book, Cyclopædia of Commercial and Business Anecdotes, to describe how banker Sir Henry Furnese profited by receiving and acting upon information about his environment before his competitors. In 1958, IBM researcher Hans Peter Luhn further developed the concept by describing a business intelligence system as an “automatic system… developed to disseminate information to the various sections of any industrial, scientific, or government organisation”. The 1980s and 1990s saw the development of DSS and the emergence of BI tools from companies such as SAP and Oracle, focusing on creating dashboards and reporting mechanisms for improved decision-making. Today, BI continues to evolve, building on analytics to support structured business decision-making processes and enabling organisations to gain competitive advantages through data-driven insights.
2.6.1.3 Artificial Intelligence
Artificial Intelligence (AI) refers to the simulation of human intelligence in machines that are programmed to perform tasks that typically require human cognition. These tasks can include learning, reasoning, problem-solving, perception, natural language understanding, and decision-making.
Artificial Intelligence began as a theoretical field in the 1940s and 1950s. Early milestones include Alan Turing’s 1950 paper, “Computing Machinery and Intelligence”, which introduced the concept of machines capable of simulating human intelligence and proposed the Turing Test as a criterion for machine intelligence. The term AI was officially coined at the 1956 Dartmouth Conference. This event is considered the birth of AI as a distinct field of study. Progress in AI was initially slow, facing periods of reduced funding and interest known as “AI winters”. However, advancements in computing technology during the late 20th century, particularly in the 1980s and 1990s, revitalised the field. The development of machine learning algorithms increased computational power, and the availability of large datasets contributed to this revival. In recent years, AI has experienced significant growth, with advancements in deep learning, natural language processing, and robotics, leading to applications across various industries, including healthcare, finance, and transportation.
2.6.1.4 Data Science
Data Science is an interdisciplinary field that focuses on extracting knowledge and insights from typically large data sets to solve problems across various application domains. It encompasses preparing data for analysis, formulating data science problems, analysing data, developing data-driven solutions, and presenting findings to inform high-level decisions.
The term Data Science has been attributed to various origins. In 1974, Peter Naur used the term in his book “Concise Survey of Computer Methods”, defining it as “the usefulness of data and data processes derives from their application in building and handling models of reality”. The field became more distinct in the early 2000s, driven by the big data revolution and the increasing need to analyse and interpret vast amounts of data. Data Science combines elements of statistics, AI, and computer science, focusing on extracting insights and building predictive models. It integrates aspects of analytics, BI, and AI to address modern data challenges, offering a comprehensive framework for working with complex datasets. The evolution of Data Science has been marked by significant milestones, including the development of machine learning algorithms, advancements in data processing technologies, and the emergence of data visualisation techniques. These developments have enabled Data Science to become a critical component in decision-making processes across various industries, from healthcare to finance.
Figure 2.5 shows the timeline summary of the evolution of key concepts in data-driven decision-making, including Analytics (1880–1940), Artificial Intelligence (1940–1970), Data Science (1970–1980), and Decision Support Systems (1980–1990), highlighting their major milestones and contributions to modern practices.
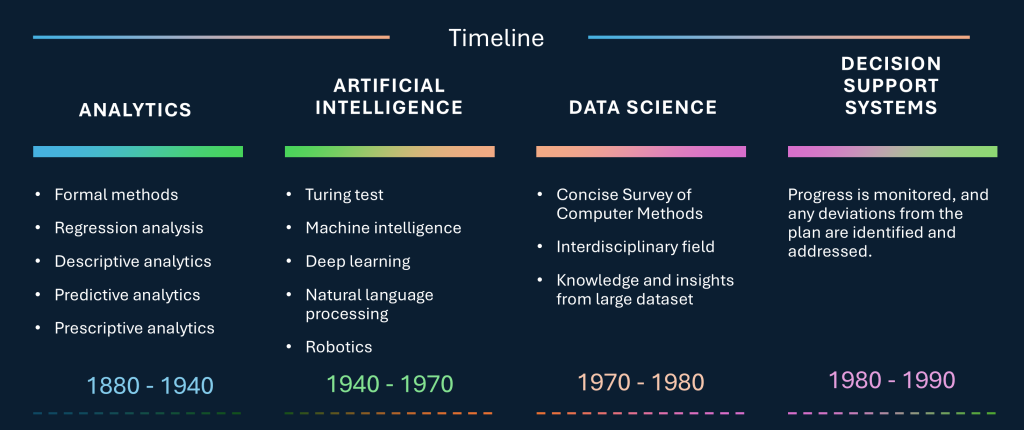
Alternative Text for Figure 2.5
A timeline summarising the evolution of data-driven technologies, spanning from Analytics (1880–1940) with regression and descriptive methods, Artificial Intelligence (1940–1970) with advancements in machine learning and robotics, Data Science (1970–1980) focusing on insights from large datasets, to Decision Support Systems (1980–1990) monitoring and addressing deviations in plans.
2.6.2 Primary purpose and focus
Table 2.3 outlines the primary purpose and focus of each field, along with the key questions they address. This comparison provides a clear understanding of how analytics, BI, AI, and data science differ in their objectives and the types of insights they deliver.
Field |
Primary Purpose |
Key questions addressed |
Analytics |
Interpreting past and present data to identify trends and patterns. |
What happened? Why did it happen? |
BI |
Organising and visualising data for operational and strategic decisions. |
What is happening now? |
AI |
Automating tasks and mimicking human intelligence. |
How can tasks be automated or decisions optimised? |
Data Science |
Solving complex problems and building predictive models. |
What patterns exist? |
2.6.3 Key features and tools
Analytics involves tools such as spreadsheets, SQL, PostgreSQL, Mongo DB, and statistical software e.g., SAS or R, which help derive descriptive and diagnostic insights. A notable Australian example is the analysis of retail sales trends by Woolworths Group to identify growth areas and customer preferences using historical transaction data.
Business Intelligence utilises dashboards, Extract-Transform-Load (ETL) processes, and BI software such as Qlik, Tableau and Power BI. For instance, the Australian financial services company ANZ Bank uses BI to create real-time performance dashboards, enabling managers to monitor key operational metrics and make data-driven decisions effectively.
Artificial Intelligence employs machine learning frameworks such as TensorFlow and PyTorch to create automated systems and predictions. An Australian application includes the use of AI in mining by companies such as Rio Tinto, which deploy autonomous vehicles and predictive maintenance systems to enhance efficiency and safety in mining operations. Additionally, AI-powered chatbots are increasingly used by Australian companies such as Qantas to provide 24/7 customer support, answering queries about flights and bookings efficiently. AI-driven recommendation engines, like those used by e-commerce platforms in Australia, personalise product suggestions based on user behaviour to enhance customer satisfaction and drive sales.
Data Science relies on programming languages such as Python and R, alongside big data platforms such as Hadoop and Spark, to develop predictive models and actionable insights. In Australia, CSIRO’s Data61 uses data science to create models that predict bushfire risks, combining environmental data and advanced analytics to support emergency planning and response. Another example is the use of data science by telecommunications companies such as Telstra, which builds predictive models to identify potential customer churn. By analysing customer behaviour patterns, Telstra can proactively address concerns and offer tailored services to improve customer retention.
2.6.4 Role in decision support
Table 2.4 compares how analytics, BI, AI, and data science contribute to decision-making processes. It highlights the level of automation in each field and the extent to which they directly or indirectly support human decisions, providing clarity on their unique roles in guiding or automating decisions.
Field |
Decision support role |
Level of automation |
Analytics |
Direct support through trend and pattern analysis |
Mostly human-driven |
BI |
Direct support via dashboards and real-time insights |
Semi-automated dashboards |
AI |
Indirect support; often automates decisions rather than supporting them |
Highly automated |
Data science |
Indirect support by providing models and patterns |
Mix of automation and human-driven exploration |
2.7 Test your knowledge
References and additional readings
-
- Alter, S. (1977). A taxonomy of decision support systems. Sloan Management Review (pre-1986), 19(1), 39.
- Foote, K. D. (2021, October 16). A brief history of data science. DATAVERSITY. Retrieved from https://www.dataversity.net/brief-history-data-science/
- Gorry, G. A., & Scott Morton, M. S. (1971). A framework for management information systems.
- Guimaraes, T., Igbaria, M., & Lu, M. T. (1992). The determinants of DSS success: An integrated model. Decision Sciences, 23(2), 409-430.
- Sharda, R., Delen, D., and Turban, E. Business Intelligence, Analytics and Data Science: A Managerial Perspective, Fourth Edition, Global Edition, Pearson Education Limited, 2018.
- Sprague Jr, R. H. (1980). A framework for the development of decision support systems. MIS quarterly, 1-26. Retrieved from:
https://citeseerx.ist.psu.edu/document?repid=rep1&type=pdf&doi=618e96a432e22e2fe353274b45d397b71a7b9ec8
Acknowledgements
Thank you to Khadeejah Atif who contributed to the design of Figure 2.2 used in this chapter.
- Decision matrices are tables that help compare different options against key criteria. For example, when choosing a supplier, a company might use a matrix to rate suppliers on cost, delivery speed, and quality, assigning scores to each and selecting the highest-rated option. ↵
- Optimisation algorithms are mathematical methods used to find the best solution from a set of options. Some commonly used optimisation algorithms are Linear Programming (LP), genetic algorithms etc. For instance, a logistics company might use an algorithm to determine the most efficient delivery routes that minimise fuel costs and travel time. ↵
- An API is a set of rules and protocols that allows different software applications to communicate with each other. It acts as an intermediary, enabling one system to access the functionality or data of another system in a structured and secure way. APIs are commonly used in web development, mobile apps, cloud computing, and various other fields to integrate services, retrieve data, or automate processes. ↵