Data to Knowledge Continuum
Amara Atif
Learning Objectives
By the end of this chapter, you will be able to:
LO1 Define data and distinguish it from information, knowledge and wisdom while exploring the progression from raw data to actionable insights.
LO2 Recognise the role of data in supporting business decisions across strategic, tactical and operational levels.
LO3 Describe the taxonomy of data, including its classification and types.
LO4 Understand the concept of analytics-ready data and the importance of data quality metrics for accurate and effective analysis.
LO5 Explore the process of data pre-processing, detailing its sub-tasks and methods with real-world examples from various industries.
1 Foundations of data: Building the basics for analytics and business intelligence
This chapter provides an overview of the fundamentals of data as a basis for analytics and business intelligence. First, it defines data and distinguishes it from information, knowledge and wisdom by describing the process that starts with raw data and ends with actionable insight. Then, it covers the role that data plays in supporting business decisions and how it impacts the firm at the strategic, tactical and operational levels. The chapter introduces a simple taxonomy of data by discussing data classification and types – this will help in building a clear understanding of its structure and usage. Further, the chapter discusses analytics-ready data, with a major focus on data quality metrics, something that becomes essential to ensure correct and effective analysis. It then goes on to cover data pre-processing, its most important sub-tasks and methods, using practical examples for various industries to illustrate the essential role it can play in representing data ready for meaningful insights.
Understanding these concepts is important because data forms the foundation of all analytics and business intelligence processes. Without a clear grasp of how to structure, assess, and prepare data effectively, organisations risk making decisions based on incomplete, low-quality, or misleading insights – something no business can afford in today’s data-driven world.
1.1 The nature of data
1.1.1 Data
The term data (singular: datum) refers to a collection of raw, unprocessed facts, measurements or observations.
Data can take many forms, including numbers, text, symbols, images, audio, video, and more. In its original form, data is unorganised and lacks context, making it difficult to interpret without further processing. Data represents the most basic level of abstraction, from which higher levels, such as information, knowledge and wisdom, are developed.
Data is classified based on how it is organised and stored (structured, semi-structured or unstructured), the types of information it represents (quantitative or qualitative), its purpose (primary or secondary) and origin/source (internal, external or generated data). For more on how data is classified, see Section 1.3 on the taxonomy of data.
1.1.2 From data to information
Information is data that has been processed, organised or structured to provide meaning and context, making it more understandable and relevant to specific purposes.
Unlike raw data, information serves a particular goal, often answering specific questions or helping with decision-making.
For example, a report summarising monthly sales totals, a demographic profile of customers or a chart illustrating website traffic over time takes raw data and organises it into a meaningful format. This organised data can now provide insights that address business questions and support objectives.
In business, converting raw data into information allows organisations to identify trends, make comparisons and gain insights that guide tactical and operational decisions.
1.1.3 From information to knowledge
Knowledge is created by analysing and synthesising information to identify patterns, relationships or insights that contribute to a deeper understanding.
It connects various pieces of information, enabling interpretation based on experience, expertise or reasoning. Knowledge goes beyond basic facts, providing meaningful insights that often lead to actionable recommendations for strategic decisions.
For example, recognising that a particular demographic consistently purchases a specific product or identifying a seasonal trend in sales data illustrates how knowledge can be used to understand customer behaviour patterns. This understanding informs strategic decisions, such as adjusting marketing efforts or planning inventory to meet customer needs.
In daily life, knowledge might be applied when someone notices that they feel more energised after a good night’s sleep and chooses to adjust their bedtime to improve daily energy levels.
1.1.4 From knowledge to wisdom
Wisdom represents the highest level in the progression from data to decision-making. It is the ability to make sound decisions and judgements based on accumulated knowledge and experience.
Wisdom involves applying knowledge with insight, ethics and a long-term perspective, carefully weighing the potential impacts of decisions. It balances short-term benefits with long-term sustainability, guiding actions that are both effective and responsible.
For example, a business leader might use historical knowledge and ethical considerations to develop a sustainable strategy that respects both profitability and environmental impact. This could involve implementing policies that foster customer trust or making decisions that balance profit with societal well-being.
In daily life, wisdom is reflected in choices such as investing time in developing a lifelong skill rather than seeking a short-term reward. Wisdom is about knowing not only “what” to do, but also “why” and “how” to make decisions that lead to positive, long-term outcomes.
1.1.5 The Data-Information-Knowledge-Wisdom (DIKW) Hierarchy
This progression from data to wisdom is commonly referred to as the DIKW hierarchy (Ackoff, 1989). Over the years, the DIKW theory has evolved and has been applied across various disciplines. In the field of business intelligence, it highlights the transformation of raw data into meaningful insights, which ultimately support wise and informed decision-making. Data serves as the foundational building block – once processed, it becomes information. When this information is analysed and synthesised, it evolves into knowledge. Finally, when knowledge is applied with ethical and long-term considerations, it becomes wisdom. Each stage in this hierarchy adds value, turning raw, unorganised facts into powerful tools for insightful and responsible decision-making that drives business success.
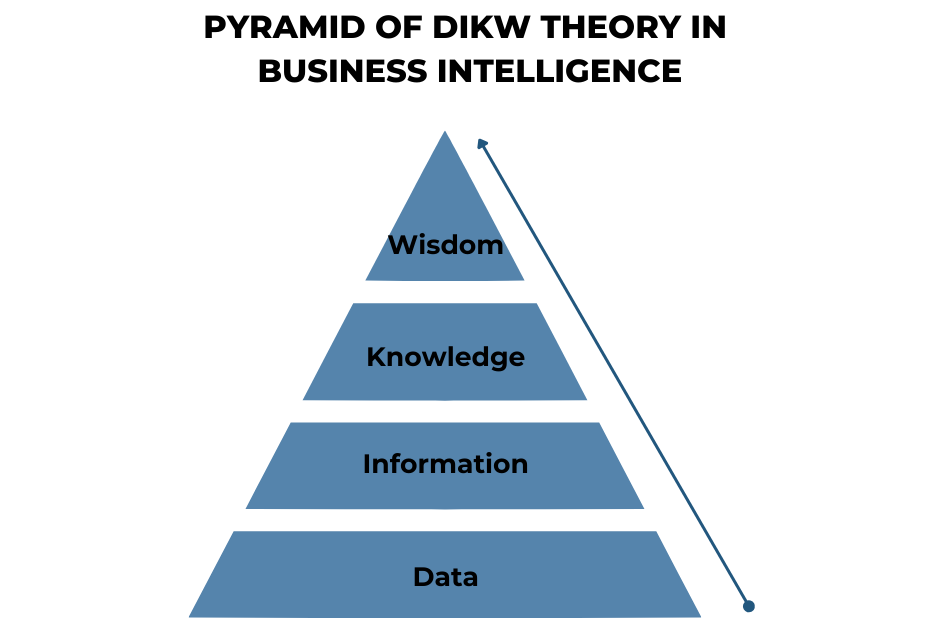
Alternative Text for Figure 1.1
The Classic DIKW pyramid shows the upwards progression of ‘Data’ at the bottom of the pyramid, to the next level which is ‘Information’, followed by ‘Knowledge’ and finally ‘Wisdom’.
1.1.6 Role of data, information, knowledge and wisdom in business intelligence
Below are two business scenarios from different industries that demonstrate how data, information, knowledge and wisdom are used in the analytics process to enhance decision-making. Scenario 1 describes how improving customer experience in retail is essential for building loyalty and satisfaction through personalised services and streamlined operations.
Scenario 1: Enhancing customer experience in a retail chain
A large retail chain wants to improve its in-store and online customer experience to boost customer satisfaction and loyalty. They decide to use analytics to understand customer behaviour, identify improvement areas and make strategic adjustments.
- Data: The retail chain collects a wide range of raw data, including transaction records, customer demographics, website click-through rates and social media engagement. They also gather customer feedback from surveys and in-store feedback forms. This data forms the foundation, providing detailed, unprocessed facts about customer behaviours and interactions.
- Information: The analytics team processes and organises this data into meaningful insights. They create reports summarising key metrics, such as popular products, peak shopping times and regional purchasing patterns. Additionally, they analyse survey responses to identify trends in customer preferences, such as a demand for eco-friendly products or faster checkout processes. This organised information helps them understand what customers are buying, when, and what preferences or pain points they have.
- Knowledge: By analysing the information, the retail chain gains knowledge about customer behaviour patterns and preferences. They observe, for example, that customers in urban areas prefer sustainable product options and that online shoppers abandon carts more frequently when shipping costs are high. This knowledge allows them to make data-driven decisions, such as adjusting product offerings in specific locations or creating promotions for online shoppers to reduce cart abandonment.
- Wisdom: The leadership team uses this knowledge to make strategic, ethically sound decisions that align with the company’s values of sustainability and customer satisfaction. They decide to introduce a loyalty program that rewards customers for eco-friendly purchases, balancing short-term profit with long-term brand loyalty and environmental impact. Additionally, they opt to subsidise shipping costs in regions with high cart abandonment rates, prioritising customer experience over immediate revenue gains. These decisions reflect wisdom by considering not only profitability but also long-term customer trust and brand reputation.
In this scenario, data provides the foundational facts, information organises these facts into trends and patterns, knowledge enables actionable insights about customer behaviour, and wisdom guides strategic decisions that align with both business goals and ethical considerations. Together, these elements allow the retail chain to improve customer experience in a way that is both effective and aligned with their brand values.
Scenario 2 describes how enhancing patient care in hospitals involves leveraging advanced technologies and efficient workflows to ensure better outcomes and a compassionate healthcare environment.
Scenario 2: Improving patient care in a hospital
A hospital wants to enhance patient outcomes and streamline its operations to deliver faster, more efficient care. To achieve this, it uses analytics to understand patient needs, optimise resource allocation and guide strategic decisions.
- Data: The hospital collects a wide range of raw data, including patient demographics, medical histories, vital signs, lab test results and feedback from post-treatment surveys. Operational data, such as wait times in the emergency room, bed occupancy rates and staff schedules, are also gathered. This raw data serves as the foundation, capturing detailed and unprocessed information about both patient care and hospital operations.
- Information: The analytics team organises and processes this data to provide insights that are more actionable. They create reports that highlight patterns, such as the average wait times for different departments, peak admission times and common health issues in certain demographics. They also analyse feedback from patients to identify areas for improvement, such as communication during treatment or responsiveness of medical staff. This organised information provides a clearer picture of hospital performance and patient needs.
- Knowledge: By analysing the information, the hospital gains knowledge about operational inefficiencies and patient care patterns. For example, the team discovers that wait times are highest in the emergency room during certain hours and that certain patient demographics are more likely to require readmission. This knowledge enables hospital administrators to make informed decisions, such as allocating more staff during peak hours in the emergency room or developing post-treatment plans for high-risk demographics to reduce readmissions.
- Wisdom: Hospital leadership uses this knowledge to make ethical, strategic decisions that balance patient care with operational efficiency. Recognising the importance of equitable care, they decide to increase staffing and resources during peak hours to reduce emergency room wait times, even if it means higher short-term costs. They also implement a patient follow-up program for high-risk groups to provide proactive care and reduce readmissions, prioritising patient well-being over immediate financial gains. These decisions reflect wisdom, as they consider both the hospital’s mission to provide quality care and the long-term benefits of improved patient satisfaction and health outcomes.
In this scenario, data provides the raw details about patients and operations, information organises this data to reveal patterns, knowledge offers actionable insights for targeted improvements, and wisdom guides strategic decisions that align with the hospital’s values and long-term objectives. Together, these elements enable the hospital to enhance patient care, optimise resources and fulfil its mission responsibly and sustainably.
1.2 Overview of data’s role in business decisions
1.2.1 Importance of clean, structured and analytics-ready data
Data is powerful only when it is prepared effectively for analysis. Raw data, while valuable, often contains noise, inconsistencies and missing information that can mislead/skew the results if not addressed. Clean, structured and analytics-ready data is essential for accurate analyses, informed decisions and meaningful business insights. Without proper data preparation, businesses risk making decisions based on incorrect or incomplete information, which can result in less effective strategies and missed opportunities.
1.2.2 Data as the foundation for business intelligence
For any organisation to leverage business intelligence tools and analytics effectively, it must first establish a robust foundation of analytics-ready data. Business intelligence systems rely on data to generate visualisations, reports and dashboards that provide managers and executives with a quick, accurate snapshot of performance.
1.2.3 The shift to data-driven decision-making
Traditionally, business decisions were often made based on intuition, past experiences or anecdotal evidence. However, with the rise of big data and analytics, there has been a shift towards data-driven decision-making, where objective insights derived from data guide strategic choices. This approach allows organisations to minimise biases, improve accuracy and make more reliable predictions about the future.
Data-driven decision-making is now widely adopted across various sectors, allowing companies to harness their data to understand customer preferences, identify trends, optimise operations and drive innovation. By incorporating data insights into the decision-making process, businesses are better equipped to respond to market changes and consumer demands with agility and confidence.
1.2.4 How data drives business insights
Figure 1.2 illustrates how data analytics drives business success across key areas in the retail industry, including trend identification, future outcome prediction, process optimisation and customer experience enhancement.
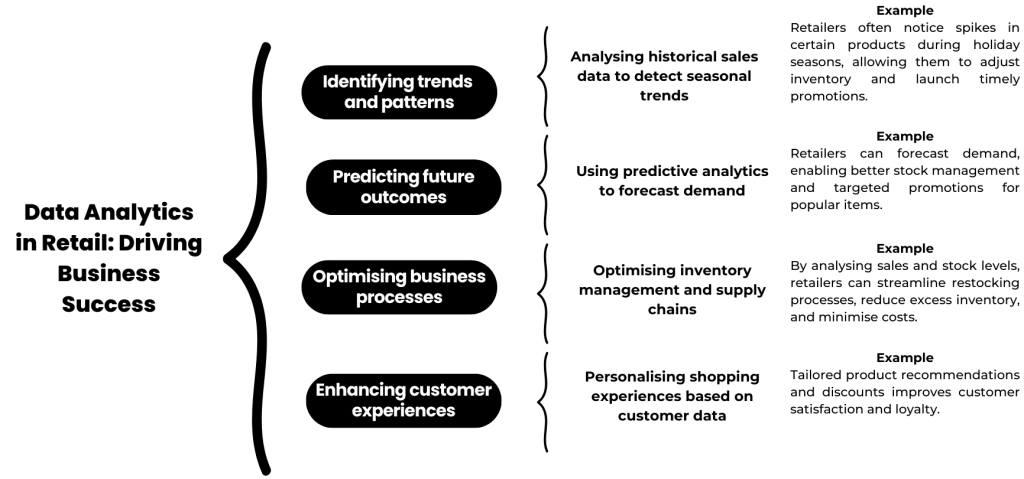
Alternative Text for Figure 1.2
Identifying trends and patterns: Data analytics helps businesses detect patterns in consumer behaviour, operational efficiency and market dynamics. For example, analysing historical sales data may reveal seasonal trends, helping companies adjust their inventory and marketing strategies accordingly.
Predicting future outcomes: Predictive analytics uses data from the past and present to forecast future trends, risks and opportunities. For instance, by analysing data on customer purchasing habits, businesses can predict which products are likely to be popular in the future, enabling proactive stock management and targeted marketing.
Optimising business processes: Data analytics supports process optimisation by identifying inefficiencies, bottlenecks or areas for improvement. In manufacturing, data from production lines can help reduce downtime and enhance quality control, while in customer service, analytics can streamline workflows, reducing response times and improving satisfaction.
Enhancing customer experiences: Data allows businesses to gain a deep understanding of customer preferences, behaviours and needs. Through data analytics, companies can personalise marketing efforts, recommend products based on customer history and improve customer service, all of which enhance the overall customer experience.
1.2.5 The role of data in driving competitive advantage
Organisations that effectively use data gain a significant competitive edge. By turning raw data into actionable insights, businesses can anticipate trends, adapt to market changes and make quicker, better-informed decisions than their competitors. In Australia, companies across various industries are using data analytics to drive success and innovation, focusing on improving customer experiences, streamlining operations (making business processes more efficient by reducing unnecessary steps, cutting down on waste and improving workflow) and developing better products.
Examples of data-driven competitive strategies in Australian industries
The examples below from Australian industries demonstrate the strategic value of data in enhancing customer personalisation, operational efficiency and product development. By integrating data analytics into core operations, Australian companies are better positioned to adapt, innovate and lead within their markets.
Customer personalisation: Australian retailers, such as Woolworths and Coles, use customer data from loyalty programs to recommend products based on previous purchases, tailor promotions and improve customer satisfaction. Woolworths, for instance, uses its Everyday Rewards program to analyse shopping habits and offer personalised discounts, boosting both engagement and sales. Similarly, Coles uses its Flybuys program to provide targeted offers that align with customer preferences, enhancing loyalty and driving sales growth.
Operational efficiency: Australian logistics company Toll Group uses data analytics to streamline delivery routes, reduce fuel consumption and improve delivery times. By analysing delivery data, Toll can optimise its supply chain logistics, minimising operational costs while enhancing customer satisfaction through timely deliveries.
Product development: Australian telecommunications giant Telstra uses customer usage data to understand product needs, identify service improvements and introduce new features that align with customer preferences. By analysing patterns in network use and customer feedback, Telstra can improve its offerings, refine its service plans and stay competitive in a dynamic market.
1.2.6 The link between data quality and decision quality
The quality of decisions is only as good as the quality of data driving them. Inaccurate, incomplete or outdated data can lead to misguided decisions, whereas high-quality data allows reliable and actionable insights. Organisations must establish rigorous data quality standards and continuously monitor and improve their data sources to support high-stakes decision-making.
Table 1.1 demonstrates the impacts of poor data quality.
Type of impact | Impacts |
Incorrect assumptions |
Decisions based on faulty data can lead to misinterpretations of customer behaviours, misalignment with market demands or improper allocation of resources. |
Lost opportunities |
Poor data quality can hide potential opportunities, such as emerging customer segments or untapped market needs. |
Risk exposure |
In industries such as finance or healthcare, low-quality data can increase risks, leading to compliance issues or compromised customer safety. |
Context
A large retail chain, GreenBite Organics, plans to launch a new product line of organic snacks based on market research and sales data from its existing product portfolio. The success of this initiative heavily depends on the quality of the data used to make decisions about product selection, pricing and marketing strategies.
Scenario description
- Incorrect assumptions: The company relies on old sales data that shows a high demand for organic snacks in a specific region. However, if they had used updated data, they would have seen that demand for these snacks was actually decreasing. As a result, the company misunderstands what customers want and spends too much on the product for that region, ending up with unsold stock and losing money.
- Lost opportunities: Because the company’s data was not well-integrated across departments, it missed noticing a new customer group, i.e., health-conscious young professionals in cities. This group was buying organic products in small amounts, but the trend was hidden due to inconsistent data formats and was overlooked during analysis. As a result, the company did not create targeted marketing campaigns for this promising group, missing a chance to grow in a valuable market.
- Risk exposure: Mistakes in the product labelling caused by inaccurate data result in wrongly claiming that one of the snacks is allergen-free. In the health-conscious food industry, this puts the company at risk of breaking regulations and facing customer lawsuits. These issues harm the company’s reputation and lead to costly penalties.
Outcome
This scenario highlights how poor data quality can lead to incorrect decisions, missed opportunities for growth and increased risks. To address these challenges, GreenBite Organics adopts robust data quality standards, focusing on timely updates, accurate analysis and consistency across departments. By implementing these measures, the company ensures more reliable decision-making and builds a foundation for sustained business success.
1.3 The taxonomy of data
In this section, we explore the fundamental classification of data, including how it is organised and stored (structured, semi-structured or unstructured), the types of information it represents (quantitative or qualitative), its purpose (primary or secondary) and origin/source (internal, external or generated data). We also discuss data granularity, which refers to the level of detail within the data. Understanding these perspectives is important for effective data management and analysis, as they play a key role in supporting business operations, generating insights and driving analytics.
In addition to these classifications, data can also be categorised by:
- Levels of measurement: Data can be categorised by levels of measurement – nominal, ordinal, interval and ratio. Each level adds a layer of understanding to data, influencing how data values can be interpreted and analysed. These levels apply to both quantitative and qualitative data and will be explored in more detail in Chapter 4: Statistical Modelling for Business Analytics.
- Usage and role in analytics: Data can be categorised as descriptive, predictive or prescriptive, each with unique functions and applications in business intelligence and analytics. These types of data will be discussed in more detail in Chapter 4: Statistical Modelling for Business Analytics, Chapter 7: Predictive Analytics and Chapter 8: Prescriptive Analytics. By classifying data based on its role in analytics, organisations can focus their analysis to achieve specific objectives, whether it’s understanding historical trends, forecasting future events or determining optimal strategies. This approach enables businesses to make more proactive, competitive and effective decisions.
Big data and its role in the taxonomy of data
Big data is not a separate type of data in the traditional taxonomy but rather a category or characteristic that encompasses all types of data. It serves as an umbrella term that includes structured, semi-structured, unstructured, quantitative, qualitative, internal, external, primary, secondary or generated, all of which are managed and analysed at scale.
In the context of a data taxonomy, big data is better understood as a characteristic or context that describes data environments with specific attributes: high volume (large-scale datasets), high variety (diverse types of data), high velocity (rapid data generation and processing), and often lower veracity (uncertainty or inconsistency in data quality). These characteristics distinguish big data by its complexity and scale rather than by its format or origin.
Instead of being a distinct type of data, big data integrates various data structures and formats, offering a comprehensive landscape for advanced analytics and business intelligence. It highlights the challenges and opportunities of managing and analysing vast, diverse datasets in real-time or near real-time scenarios.
1.3.1 Types of data in a business context
In business, data comes in various forms, each playing an important role in helping companies make informed decisions. Data can also vary in its level of granularity or the degree of detail it captures, which affects its usability in analysis.
1.3.1.1 Structured and quantitative data
Quantitative data, being numerical and measurable, is often stored as structured data. Examples include sales figures, customer counts and revenue records, which are typically organised into rows and columns within relational databases. Structured data formats (e.g., spreadsheets and databases) facilitate easy calculation of key performance indicators (KPIs), trend tracking and statistical analysis, aligning closely with the goals of quantitative data.
Granularity further defines the level of detail in structured quantitative data. High granularity provides specific and detailed information, such as individual transaction records that include data like purchase time, items bought and customer details. This level of detail is useful for identifying precise patterns and trends. In contrast, low granularity involves aggregated data, such as monthly sales totals, which offer a broader and less detailed view. High-granularity data is ideal for deep analysis, while low-granularity data is better suited for observing overall trends and making strategic decisions. The appropriate level of granularity depends on the purpose of the analysis and the type of insights required.
1.3.1.2 Unstructured, semi-structured and qualitative data
Qualitative data, being descriptive and often non-numeric, frequently appears in semi-structured or unstructured formats. Examples for unstructured formats include images (both human- and machine-generated), video files, audio files, product reviews, social media posts, and messages sent by Short Messaging Service (SMS) or online platforms. Semi-structured formats, such as emails, combine structured metadata (e.g., headers like date, language, and recipient’s email address) with unstructured data in the email body, which contains the actual message. Additionally, qualitative data, such as customer feedback may be stored in semi-structured formats, e.g., JSON (JavaScript Object Notation) or XML (Extensible Markup Language) files.
These types of data require specialised methods, such as text mining and natural language processing (NLP), to extract meaningful insights about customer preferences, opinions and areas where processes can be improved. High-granularity unstructured data, such as individual customer comments, provides rich detail but may require extensive processing, whereas summaries or tags offer a more manageable, lower-granularity view.
1.3.1.3 Integration of data types for decision-making
Quantitative and qualitative data often overlap and complement each other, providing different perspectives that support effective decision-making. Quantitative data can exist in semi-structured or unstructured formats, such as numerical information in web analytics logs (e.g., session times or page views) or metadata in image and video files (e.g., timestamps or geolocation). While most structured data is numerical (quantitative), certain qualitative data can also be structured when categorised or coded systematically. For example, qualitative data may be embedded in structured formats, such as survey responses that combine predefined categorical answers with open-ended feedback.
When integrated, these data types create a more complete picture. Quantitative data provides measurable insights, enabling businesses to track performance and make objective comparisons, while qualitative data adds context by explaining the “why” behind the numbers. Together, they allow businesses to make informed, evidence-based decisions that are both data-driven and enriched with deeper insights.
Table 1.2 illustrates specific examples of quantitative and qualitative data across different business functions, including customer and marketing, sales and revenue, and operations and production. This table provides a clearer understanding of how various data types can support business operations, enable performance tracking and contribute to strategic decision-making.
Types of Data in Business |
Quantitative Data Examples |
Qualitative Data Examples |
Customer and marketing |
|
|
Sales and revenue |
|
|
Operations and production |
|
|
Employee and workforce |
|
|
Web and digital analytics |
|
|
Finance and compliance |
|
|
1.3.1.4 Data purpose and origin/sources
Data used in business comes from various sources, broadly categorised as primary internal, external or generated data, and secondary internal, external or generated data.
Primary data is collected firsthand by an organisation for a specific purpose. It is original and obtained directly from the source through surveys, experiments, observations or direct measurements. Primary data is often more reliable for specific analysis but can be time-consuming and costly to gather. Primary data can be internal (collected within the organisation, typically structured), external (collected from sources outside the organisation, structured or semi-structured) or generated (created through interactions or automated systems, structured, semi-structured or unstructured).
Secondary data refers to data collected by a third party, often for general purposes, which can be reused in other contexts. Secondary data is often more accessible and affordable than primary data but may be less tailored to specific analytical needs. Using secondary data saves time and resources by reusing existing data to gain insights. Like primary data, secondary data can also be internal (structured or semi-structured), external (typically semi-structured or unstructured) or generated (can be structured, semi-structured or unstructured).
Internal data is collected and generated within an organisation’s own systems, processes or operations. It originates from the organisation’s activities and is typically exclusive to the organisation.
External data is acquired from sources outside the organisation. This data provides insights into external factors that impact the business, such as market conditions, industry trends and customer behaviour outside direct interactions with the organisation.
Generated data is created as a result of interactions, activities or usage patterns, and can be internal or external. Generated data may come from various sources, including user interactions, sensor readings or automated systems. It can be structured, semi-structured or unstructured and offers unique insights into behaviours and performance.
The data classification matrix in Table 1.3 illustrates these categories and their relationships, making it easy to understand how these data types overlap and interact.
Aspect |
Primary Internal Data |
Primary External Data |
Definition |
Data collected within the organisation |
Data collected by the organisation from external sources |
Use |
Used for operational decisions, performance monitoring and process improvements |
Used to understand customer needs, market trends and external factors for strategic planning |
Examples |
|
|
|
Secondary Internal Data |
Secondary External Data |
Definition |
Previously collected internal data repurposed (reused) |
Data collected by other organisations or entities |
Use |
Useful for trend analysis, long-term planning and optimising existing processes |
Provides broader market insights and benchmarking and supports strategic decision-making without primary collection |
Examples |
|
|
1.3.2 Data as a strategic asset
Data is often referred to as the “new oil” because it is essential to driving competitive advantage. Data-driven companies use insights derived from data to make informed decisions, improve products, optimise operations and personalise customer experiences. Businesses depend on data to guide strategic, tactical and operational decisions, using it to reduce uncertainty and provide evidence-based support for selecting the best course of action.
The following real-world example illustrates how Australian retailers treat data as a strategic asset to enhance their competitiveness and customer satisfaction.
In Australia’s retail industry, leading companies such as Woolworths, Coles, IGA and Aldi all leverage data as a strategic asset to enhance competitiveness and improve customer satisfaction. These retailers collect extensive data from loyalty programs, online shopping behaviours and in-store purchases, enabling them to track customer preferences, seasonal trends and regional demand patterns. By analysing this data, they can make informed decisions at all levels – from strategic to operational.
At the strategic level, Australian retailers use data insights to identify and respond to market trends, such as the growing demand for organic products and sustainable packaging. For example, Woolworths and Coles have expanded their organic product ranges and environmentally friendly options, positioning themselves as leaders in sustainable retail.
At the tactical level, data helps retailers plan regional promotions tailored to customer preferences. For instance, during the holiday season, stores in warmer areas might promote outdoor products and summer foods, while stores in cooler regions stock up on winter essentials. This targeted approach allows retailers to connect more effectively with local customers.
At the operational level, data on customer transactions helps these retailers optimise inventory management, ensuring popular items are consistently available while minimising overstock. By using predictive models, they can anticipate demand surges for specific products and adjust stock levels, accordingly, reducing waste and improving the customer experience.
Through these data-driven practices, Australian retailers like Woolworths, Coles, IGA and Aldi enhance customer personalisation, streamline operations and adapt to shifting market trends, giving them a competitive edge. This example demonstrates how data, when treated as a strategic asset, empowers these companies to make evidence-based decisions that drive growth, foster customer loyalty and maintain their market positions in Australia’s retail sector.
1.4 Metrics for analytics-ready data
In this section, we explore the essential metrics that define analytics-ready data, highlighting the qualities that make data reliable, meaningful and suitable for analysis. Analytics-ready data is crucial for organisations that aim to derive insights effectively and efficiently, as it minimises the risk of errors and maximises the value extracted from data.
1.4.1 Definition of analytics-ready data
What are analytics-ready data? Analytics-ready data refers to data that has been prepared to meet the requirements of analysis. This means the data is clean, consistent, complete and formatted in a way that allows for easy interpretation. To achieve this state, the data undergoes pre-processing steps, such as cleaning, transformation and validation, to align it with the specific goals of the analysis.
Why is analytics-ready data important? Having analytics-ready data saves time, reduces errors and enables quicker insights by providing a solid foundation for analysis. Without proper data quality measures in place, analysts risk incomplete results, inaccuracies or misinterpretations, potentially leading to costly business decisions based on flawed data.
1.4.2 Data quality metrics
Data quality is essential to ensure the accuracy, reliability and value of analytics. High-quality data supports effective decision-making, minimises errors and enhances the overall impact of analysis. Below are the key metrics for analytics-ready data, each explained with examples. Here is a simple poem for you that was generated using Copilot (Microsoft, 2024).
Reliable and complete, data stands tall,
Consistent and accurate, the key to it all.
Timely and valid, with uniqueness in play,
Accessible and rich to brighten the day.
Diverse and traceable, it tells the tale,
Interoperable, scalable, it will never fail.
Relevance and context give it its might,
Granularity and integrity keep it tight.
Secure and private, it passes the test,
With interpretability, it’s simply the best!
1.4.2.1 Data reliability
Reliability refers to the consistency and dependability of data over time and across processes or systems, ensuring unbiased and accurate information regardless of when or where it is collected or used.
Impact on analytics: Unreliable or inaccurate data can lead to misleading insights and flawed decisions.
Examples:
- A hospital depends on dependable readings from medical devices (e.g., heart rate monitors) to ensure accurate diagnosis and treatment. If the data fluctuates or becomes unreliable due to sensor malfunctions, it can compromise patient safety.
- A retailer analysing weekly sales data needs consistency in how sales are recorded across different stores and systems. If some stores log discounts differently or omit promotions, the data becomes unreliable for decision-making.
1.4.2.2 Data consistency
Consistency means that data values remain uniform (identical and accurate) across datasets and systems.
Impact on analytics: Inconsistent data creates confusion and reduces trust in analytics results.
Example: If a customer’s address is updated in a customer relationship management (CRM) system, the same updated address should appear in the billing system and any associated databases. If the CRM shows the new address while the billing system still shows the old one, the data is inconsistent, which could lead to billing errors or delivery issues.
1.4.2.3 Data completeness
Completeness refers to the extent to which all required data fields, including the metadata that describes the characteristics and context of the data, are available and properly filled.
Impact on analytics: Incomplete/missing data can lead to gaps in analysis and poor decision-making, as critical insights may be overlooked or misinterpreted due to missing information.
Examples:
- A retail business must ensure all customer profiles include essential details such as name, email and purchase history to personalise marketing campaigns effectively.
- A hospital requires complete patient medical records, including allergies and medication history, to avoid treatment errors and improve patient safety.
- Metadata for a dataset of images might include details such as file size, resolution, timestamps and descriptive tags, which provide additional context and improve the ability to retrieve, analyse and interpret the data.
1.4.2.4 Data content accuracy
Accuracy ensures that data values are correct and reflect the true state of the object or event they represent.
Impact on analytics: Inaccurate data can lead to false conclusions, impacting business strategies and operational processes.
Examples:
- An airline reservation system must accurately capture flight schedules and passenger bookings to avoid overbooking.
- Sensor data from IoT devices used in agriculture must correctly measure soil moisture levels to optimise irrigation systems.
1.4.2.5 Data timeliness and temporal relevance
Timeliness (also referred to as data currency) ensures that data is up-to-date and available at the moment when it is needed for decision-making.
Temporal relevance ensures that data corresponds to the appropriate time frame for the specific analysis or decision-making process.
Impact on analytics: Outdated data makes insights irrelevant and can lead to missed opportunities. Using data from an inappropriate time period can result in incorrect conclusions, as it may no longer reflect the current or relevant conditions. Together, these metrics ensure decisions are based on both the most current data and data that is contextually appropriate for the situation.
Examples:
- A logistics company needs real-time tracking data to monitor delivery statuses and optimise routes.
- A retail business should rely on recent sales data to plan seasonal promotions, rather than outdated figures from prior years.
1.4.2.6 Data validity
Validity ensures that data follows predefined formats, types or business rules.
Impact on analytics: Invalid data can disrupt processes and analytics workflows.
Examples:
- A payroll system requires dates in a valid format (e.g., MM/DD/YYYY) to process employee salaries correctly.
- Customer phone numbers in a CRM system must match a specific format (e.g., country code included) to enable seamless communication.
1.4.2.7 Data Uniqueness
Uniqueness means that no duplicate records exist within a dataset.
Impact on analytics: Duplicate data can inflate metrics, skew results and increase storage costs.
Examples:
- An email marketing platform must ensure that each email address in its database is unique to avoid sending multiple messages to the same recipient.
- A university admissions system must ensure that no duplicate student records are created during the application process.
1.4.2.8 Data accessibility
Data accessibility refers to the ease with which authorised users can locate, retrieve and use data when needed for analysis or decision-making.
Impact on analytics: Poor accessibility can delay operations, hinder decision-making and reduce the effectiveness of analytics.
Examples:
- A doctor accessing a patient’s electronic health records (EHR) during an emergency needs quick and seamless access to critical medical data, such as allergies and previous treatments, to make life-saving decisions.
- A logistics company relies on real-time accessibility of tracking data to monitor shipments, reroute deliveries or notify customers of delays.
1.4.2.9 Data usability
Data usability is the ease with which data can be accessed, understood and utilised by end-users.
Impact on analytics: Complex or poorly organised data may hinder its use in analytics, reducing the effectiveness of insights.
Example: A dashboard that provides easy access to interactive data visualisations ensures users can quickly explore trends and make decisions.
1.4.2.10 Data integrity
Data integrity ensures that relationships between data elements are maintained correctly.
Impact on analytics: Data with broken relationships can result in incomplete or misleading analyses.
Examples:
- In a relational database, foreign key relationships between order details and customer information must remain intact for accurate reporting.
- A supply chain management system must ensure that product IDs in the inventory table match those in the supplier table.
1.4.2.11 Data richness
Data richness refers to the depth, breadth and diversity of data available for analysis.
Impact on analytics: Lack of rich data restricts the ability to gain meaningful insights and can result in inefficient strategies or actions.
Examples:
- A hospital collects rich data about patients, including demographics, medical history, lab results, prescriptions and lifestyle habits to create personalised treatment plans and predictive models for patient care.
- An e-commerce platform gathers data on customer browsing behaviour, purchase history, product reviews and payment preferences to create personalised recommendations.
1.4.2.12 Data diversity
Data diversity refers to the variety of data types and sources, ensuring a multi-dimensional view.
Impact on analytics: Incorporating diverse datasets (structured, unstructured, quantitative, qualitative) provides a richer, more comprehensive perspective.
Example: Combining social media sentiment (qualitative) with sales data (quantitative) enables a company to align product popularity with customer feedback.
1.4.2.13 Data interpretability
Data interpretability is the extent to which the data can be analysed and understood in a meaningful way.
Impact on analytics: Data that is too complex or poorly presented can hinder actionable insights.
Example: In machine learning models, interpretable datasets allow analysts to understand how certain variables influence predictions.
1.4.2.14 Data relevance
Data relevance refers to measures of whether the data is meaningful and applicable to the specific analysis or business question.
Impact on analytics: Irrelevant data can clutter analysis, leading to noise that distracts from actionable insights.
Example: In marketing, data on customer purchasing behaviours is relevant, but unrelated demographic data (e.g., age of non-target groups) may be less useful.
1.4.2.15 Data contextuality
Data contextuality refers to whether the data captures the context surrounding an event or transaction, enhancing its interpretability.
Impact on analytics: Data without context may lead to inaccurate assumptions.
Example: Knowing that sales spiked in a specific region is valuable, but understanding that it occurred during a festival (context) provides actionable insights for future marketing campaigns.
1.4.2.16 Data granularity
Data granularity refers to the level of detail in the data. High granularity means the data is very detailed, while low granularity provides a summarised view.
Impact on analytics: The level of detail impacts the depth of analysis; high granularity enables micro-level insights, while low granularity is better for high-level trends.
Example: In customer analytics, transaction-level data provides granular insights into individual behaviour, while monthly summaries show macro trends.
1.4.2.17 Data traceability
Data traceability is the ability to trace data back to its source and track its transformations during processing.
Impact on analytics: Traceability ensures transparency, builds trust in the analysis and enables verification of results.
Example: In financial audits, having a clear record of data origins and modifications is critical to validate outcomes.
1.4.2.18 Data interoperability
Data interoperability refers to the ability of data to integrate seamlessly across different platforms, systems and tools.
Impact on analytics: Non-interoperable data creates silos, making it harder to conduct a comprehensive analysis.
Example: A supply chain system that integrates data from logistics providers, inventory systems and customer orders enables a unified view of operations.
1.4.2.19 Data scalability
Data scalability ensures that the data infrastructure can handle growth in data volume, variety and complexity without degrading performance.
Impact on analytics: Analytics systems should be able to process increasing amounts of data as organisations grow.
Example: An IoT platform should scale to accommodate additional sensors as a factory expands operations.
1.4.2.20 Data security and data privacy
Data security refers to the measures and technologies used to protect data from unauthorised access, breaches, theft or corruption.
Data privacy refers to the rights of individuals to control how their personal data is collected, stored and shared in compliance with ethical and legal standards.
Impact on analytics: Weak data security and privacy protocols can result in financial losses, reputational damage and legal consequences.
Examples:
- Banks and financial institutions must secure customer financial records and transaction data to prevent fraud or hacking attempts. Similarly, hospitals use encryption and access controls to safeguard patient medical records, ensuring only authorised personnel can view sensitive information.
- Social media platforms, e.g., Facebook, must ensure that user data is shared only with consent, particularly for advertising purposes. Additionally, a retailer collecting customer email addresses and purchase history must comply with privacy regulations by allowing customers to opt out of marketing communications.
1.5 Data pre-processing
Data pre-processing is the process of preparing raw data for analysis by applying techniques such as cleaning, transformation, reduction and integration. It ensures that data is in an optimal state for generating reliable and accurate insights. Proper data pre-processing is essential for analytics as it enhances data quality, minimises errors and improves the performance of analytical models.
Pre-processing is a prerequisite for analysis, focusing on data preparation rather than generating insights. By cleaning, transforming, integrating and formatting the data, pre-processing ensures high-quality, well-structured and analytics-ready data, which forms the foundation for meaningful and reliable analysis.
1.5.1 Importance of pre-processing for analytics
Raw data is often messy, incomplete, inconsistent and unsuitable for direct analysis. It may contain errors, outliers and redundancies that can distort analytics and lead to inaccurate or misleading results. Effective pre-processing addresses these issues by cleaning and organising the data, making it cleaner, more consistent and ready for meaningful analysis. Without proper pre-processing, the accuracy and reliability of analytics outcomes are significantly compromised.
1.5.2 Steps in data pre-processing
Data pre-processing is a structured process that involves several sequential steps to prepare raw data for analysis. Each step is designed to address specific issues within the data, ensuring that it is clean, accurate and in a usable format for generating meaningful insights. These steps often include sub-tasks and methods tailored to meet the unique requirements of the dataset or analytical task. By following these steps, businesses can ensure that their data is of high quality and ready for reliable analysis.
Figure 1.3 describes the steps in basic data pre-processing.
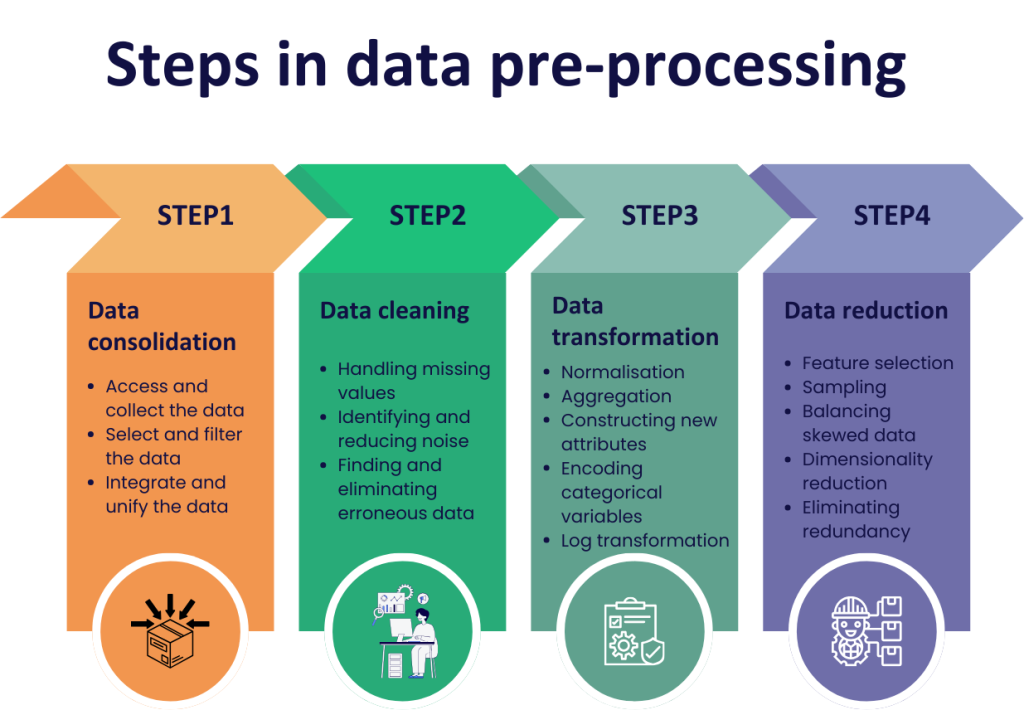
Alternative Text for Figure 1.3
Steps in Data Pre-Processing:
Step 1: Data Consolidation
- Access and collect data.
- Select and filter the data.
- Integrate and unify the data.
Step 2: Data Cleaning
- Handle missing values.
- Identify and reduce noise.
- Find and eliminate erroneous data.
Step 3: Data Transformation
- Perform normalisation.
- Aggregate data.
- Construct new attributes.
- Encode categorical variables.
- Apply log transformation.
Step 4: Data Reduction
- Select features.
- Sample data.
- Balance skewed data.
- Perform dimensionality reduction.
- Eliminate redundancy.
Each step is visually represented as a distinct arrow, highlighting the sequential nature of the process. Icons at the bottom of each arrow symbolise tasks within the respective steps.
1.5.2.1 Data consolidation
In a business setting, data consolidation is the process of combining information collected from different systems or tools into one central place. This is especially important because businesses often use multiple systems to manage different aspects of their operations. When these systems operate separately, the data they hold can become siloed, meaning it is scattered across different platforms and not easily accessible as a whole. This makes it harder for businesses to see the “big picture” or draw meaningful conclusions. By consolidating this data into one system, businesses can create a unified view of their operations, customers and performance. This unified data is easier to analyse, enabling better decision-making and more efficient business processes.
Table 1.4 provides an overview of the sub-tasks in data consolidation, along with an example for each.
Sub-task |
Description |
Example |
Access and collect the data |
This step involves locating and retrieving raw data from various sources such as databases, cloud storage, web services or even physical records. |
A retail company collects sales data from its point-of-sale (POS) system, inventory data from its warehouse system and customer feedback from social media platforms. |
Select and filter the data |
After collecting data, this step involves identifying and selecting only the relevant data for the analysis. Irrelevant or unnecessary data is removed to ensure the focus remains on actionable insights. |
A company analysing sales performance may filter out fields such as “employee birthdays” or “holiday schedules” as they are irrelevant to the sales analysis. |
Integrate and unify the data |
This step aligns data from different sources to create a consistent and unified dataset. It resolves discrepancies such as mismatched formats, different naming conventions or varying levels of granularity. |
A financial institution consolidates transaction data from multiple branches, ensuring that date formats and account names follow a uniform standard. |
Table 1.5 provides an overview of the methods involved in data consolidation, followed by a business scenario illustrating how these methods can be applied in practice.
Method Name |
Description |
SQL (Structured Query Language) queries |
SQL is widely used to extract, transform and merge data from relational databases. |
Software agents and automation tools |
Automation tools, e.g., robotic process automation (RPA) or software agents, can collect and consolidate data from multiple sources, including APIs or web services. |
Domain expertise and statistical tools |
Experts and tools are often needed to determine which data is relevant and how to align it. This includes cleaning inconsistencies during consolidation. |
Ontology-driven data mapping |
Ontology-driven methods help map data fields from different sources into a unified schema. This is particularly useful when merging datasets with different structures. |
Business scenario: Enhancing customer insights with consolidation methods
A retail company aims to improve its understanding of customer behaviour by integrating data from multiple systems, including a CRM database, an online sales platform, social media reviews and in-store transaction records. By using various consolidation methods, the company ensures that the data is consistent, comprehensive and ready for analysis.
- SQL queries for data integration
The company uses SQL queries to join customer information from the CRM database with purchase histories stored in the sales database. For example, they query customer IDs in both databases to link customer demographics (age, gender, location) with their recent purchases.
Result: This allows the company to identify purchasing patterns, such as which age group prefers specific product categories, enabling targeted marketing campaigns.
- Software agents for automation
The company implements an automated agent to collect product reviews from multiple online platforms, such as their website, social media channels and third-party review sites. The agent consolidates this data into a central database for further analysis.
Result: By analysing the consolidated reviews, the company identifies common customer concerns, such as delayed deliveries or packaging quality, and prioritises improvements.
- Domain expertise and statistical tools
Using statistical tools, domain experts examine the merged datasets to resolve inconsistencies, such as duplicate customer records or outliers in transaction amounts. For instance, they detect and correct a data entry error where a product price was mistakenly recorded as $10,000 instead of $100.
Result: This ensures that the final dataset is clean and accurate, providing reliable insights for decision-making.
- Ontology-driven data mapping for unified structure
The company faces a challenge when merging data from in-store transactions and online sales, as these systems use different terminologies (e.g., “item number” in one system vs. “product ID” in another). By applying ontology-driven data mapping, the company aligns these fields into a unified structure.
Result: This enables seamless integration, allowing the company to analyse sales performance across both online and offline channels holistically.
1.5.2.2 Data cleaning
Data cleaning is the process of identifying and correcting errors, inconsistencies and missing pieces of information in a dataset to improve its quality and reliability. This step in data pre-processing ensures that data is accurate, complete and suitable for analysis. Without cleaning, the data might contain mistakes or gaps that can lead to incorrect results and poor decision-making.
In practice, raw data collected from various sources often contains issues such as missing values, duplicate entries, incorrect formats and outliers, which must be resolved before meaningful analysis can take place.
Table 1.6 provides an overview of the sub-tasks in data cleaning, along with an example for each.
Sub-task |
Description |
Example |
Handling missing values |
Missing values occur when data points are not recorded or are unavailable. Handling missing values ensures the dataset remains usable. |
Filling in missing ages in a customer dataset using the average age of the existing entries. |
Identifying and reducing noise |
Noise refers to irrelevant or meaningless data that can obscure useful insights. Reducing noise helps clarify patterns and relationships within the data. |
Smoothing extreme spikes in stock price data that result from anomalies in the recording process. |
Finding and eliminating erroneous data |
Erroneous data includes invalid, incorrect or inconsistent values in the dataset. Identifying and correcting such errors ensures accuracy. |
Correcting product prices listed as negative due to a data entry error. |
Table 1.7 provides an overview of the methods involved in data cleaning, followed by a business scenario illustrating how these methods can be applied in practice.
Method Name |
Description |
Imputation for missing values |
Replace missing data with estimated values based on statistical techniques or machine learning models. |
Outlier detection and treatment |
Detect outliers using statistical methods (e.g., standard deviation, interquartile range) or clustering algorithms. Treat them by removing, capping or adjusting their values. |
Data de-duplication |
Identify and remove duplicate entries in the dataset to ensure uniqueness. |
Error correction tools |
Use automated tools or manual review processes to correct invalid or inconsistent data values. |
Smoothing techniques |
Address noisy data points (due to general fluctuations, which may or may not include outliers) by replacing them with averages (of surrounding records) or applying regression techniques. |
Note: Outlier detection can be part of a smoothing process. For instance, you might first detect and remove outliers and then apply smoothing to clean the dataset further.
Business scenario: Preparing healthcare data for patient outcome analysis
A hospital wants to analyse patient data to identify patterns in treatment outcomes and optimise resource allocation. However, the raw data collected from different sources, such as electronic health records (EHR), lab reports and wearable health devices, contains several issues, such as missing values, outliers, duplicates and inconsistencies. The hospital applies various data cleaning and preparation methods to create a reliable dataset for analysis.
- Imputation for missing values
Some patient records have missing values for critical measurements, such as blood pressure or glucose levels, during routine check-ups. The hospital uses mean imputation to fill in the gaps by calculating the average value for these parameters based on other patients of similar age and medical history.
Result: The completed dataset ensures that missing values do not compromise the accuracy of health trend analysis.
- Outlier detection and treatment
While reviewing wearable device data, the hospital identifies abnormally high heart rate readings for a few patients that were far outside the normal range. Using statistical methods like the interquartile range (IQR), these outliers are flagged. Upon review, some outliers are corrected (e.g., a heart rate of 600 beats per minute due to a device malfunction), while others (valid but extreme cases) are retained for further investigation.
Result: The hospital prevents incorrect conclusions caused by faulty device readings while maintaining valid data for rare cases.
- Data de-duplication
The patient database contains duplicate entries caused by multiple visits, where patients used slightly different identifiers (e.g., one visit uses their full name, another their initials). Using a data de-duplication algorithm, the hospital matches records based on attributes like patient ID, date of birth and contact information to merge duplicates.
Result: A clean, unique patient dataset enables accurate tracking of treatment histories and outcomes.
- Error correction tools
Some lab results have inconsistencies in how measurements are recorded, e.g., cholesterol levels in one system are recorded in mg/dL, while another system uses mmol/L. The hospital applies an error correction script to standardise all units to mg/dL.
Result: Standardised units ensure that comparisons across lab results are accurate and meaningful for medical analysis.
- Smoothing techniques
Wearable health devices report daily step counts for patients recovering from surgery. These reports show erratic fluctuations due to device errors or irregular recording intervals. The hospital applies a moving average smoothing technique to replace noisy values with smoothed data that represents overall activity trends.
Result: The smoothed data allows the hospital to monitor patient recovery progress and identify when additional interventions may be needed.
1.5.2.3 Data transformation
Data transformation is the process of converting raw data into a format suitable for analysis. It involves modifying the structure, format or values of data to meet specific requirements of the analytics task. Transformation ensures consistency and standardisation, allowing better compatibility with analytical tools and techniques. By applying transformation techniques, data becomes easier to interpret, analyse and apply in tasks like machine learning, statistical studies or business reporting.
Table 1.8 provides an overview of the sub-tasks in data transformation, along with an example for each.
Sub-task | Description | Example |
Normalisation | Adjusting the scale of numerical data to a common range, often between 0 and 1 or –1 and +1. This eliminates biases caused by differences in measurement units or magnitudes. | Scaling sales revenue (measured in millions) and customer ratings (measured on a scale of 1 to 5) to the same range for consistent comparison. |
Discretisation or aggregation | Converting continuous numerical data into discrete categories (discretisation) or summarising multiple data points into a single value (aggregation). | Converting customer ages into ranges, e.g., “20–30” or aggregating daily sales data into monthly totals. |
Constructing new attributes | Deriving new variables or features from existing ones by applying mathematical, logical or statistical operations. | Creating a “customer loyalty score” by combining purchase frequency, average transaction value and account age. |
Encoding categorical variables |
|
|
Log transformation | Applying a logarithmic function to data to reduce skewness or compress large ranges of values. | Transforming sales figures in millions using logarithms to reduce large disparities. |
Table 1.9 provides an overview of the methods involved in data transformation, followed by a business scenario illustrating how these methods can be applied in practice.
Method Name |
Description |
Scaling techniques |
Adjusts data values to fit within a standard range or distribution. |
Binning or discretisation |
Groups numerical data into intervals or bins. |
Mathematical transformations |
Applies functions like logarithms, square roots or exponentials to data values. |
Feature engineering |
Creates new variables by combining or modifying existing ones. |
Text transformation |
Converts unstructured text data into structured formats for analysis. |
Dimensionality reduction |
Reduces the number of features in a dataset while retaining critical information. |
Business scenario: Enhancing supply chain analytics for a manufacturing company
A manufacturing company wants to optimise its supply chain operations to reduce costs, improve delivery times and forecast demand more effectively. However, the raw data from various sources, such as supplier records, inventory logs and shipment tracking systems, requires significant pre-processing to be suitable for analysis. The company applies various data transformation methods to prepare the data for insights.
- Scaling techniques
The company’s inventory data includes a wide range of values, such as the number of units (ranging from 1 to 100,000) and shipment costs (ranging from $0.01 to $1,000). To ensure all features are comparable, the company applies Min-Max Scaling to normalise these variables between 0 and 1.
Result: Scaled data ensures that no single variable dominates the analysis due to differences in magnitude, improving the performance of predictive models for inventory optimisation.
- Binning or discretisation
The company’s demand forecast dataset includes the age of customers ordering products, which ranges widely from teenagers to seniors. To simplify the analysis, the company groups ages into bins, such as “Under 18”, “18–35” and “35+”.
Result: The binned data allows the company to analyse demand patterns for different age groups and target its marketing efforts accordingly.
- Mathematical transformations
The company’s shipment cost data is highly skewed, with a small number of unusually high costs distorting the averages. To address this, the company applies a logarithmic transformation to reduce the skewness and make the data more suitable for statistical modelling.
Result: Transformed data provides more meaningful insights into average shipment costs and highlights areas where cost optimisation is needed.
- Feature engineering
The company created a new feature, “inventory turnover rate”, by dividing the total number of units sold by the average inventory level. This variable helps measure how efficiently inventory is managed across different product categories.
Result: The new feature enables the company to identify slow-moving products and adjust its inventory strategy to reduce holding costs.
- Text transformation
Supplier feedback forms contain unstructured text data describing delays, quality issues and other concerns. The company applies text transformation techniques such as TF-IDF (Term Frequency-Inverse Document Frequency) to extract keywords and convert the text into numerical representations.
Result: The structured text data enables the company to identify common supplier issues and prioritise improvements in supplier relationships.
- Dimensionality reduction
The dataset includes over 50 variables related to shipment tracking, such as delivery times, route distances, vehicle types and fuel costs. Many of these features are highly correlated. The company uses Principal Component Analysis (PCA) to reduce the dataset to a smaller set of uncorrelated components while retaining the majority of the original information.
Result: Dimensionality reduction simplifies the dataset, making it easier to build machine learning models that predict delivery delays or cost overruns.
1.5.2.4 Data reduction
Data reduction is the process of making large datasets smaller and simpler without losing important information. The goal is to reduce the size, complexity or number of variables in the data while keeping its key insights and usefulness intact. Data reduction helps improve the speed and efficiency of analysis, lowers storage costs and removes unnecessary “noise” from the data, making it easier to focus on meaningful insights.
Large datasets often include extra or irrelevant information that can overwhelm analysis tools and waste resources. Data reduction ensures that only the most important parts of the data are kept, helping analysts focus on the variables and records that truly matter. This makes the analytics process faster, more efficient and easier to manage.
Table 1.10 provides an overview of the sub-tasks in data reduction, along with an example for each.
Sub-task |
Description |
Example |
Reducing the number of attributes (feature selection) | Identifying and retaining only the most relevant variables (attributes) for analysis. | In predicting customer churn, variables such as “purchase frequency” and “customer satisfaction score” might be more relevant than “store location”. |
Reducing the number of records (sampling) | Selecting a representative subset of the dataset while discarding redundant or unnecessary records. | A bank may analyse a sample of transactions rather than the entire dataset to detect fraud trends. |
Balancing skewed data | Adjusting datasets with imbalanced classes to ensure fair representation of all categories. | In fraud detection, oversampling fraudulent transactions ensures the model recognises minority-class patterns effectively. |
Dimensionality reduction | Reducing the number of features or dimensions while retaining the dataset’s essential characteristics. | Combining highly correlated features (e.g., “height” and “weight”) into a single dimension using Principal Component Analysis (PCA). |
Eliminating redundancy | Removing duplicate or irrelevant records and attributes. | A CRM system might eliminate duplicate customer profiles to streamline marketing efforts. |
Table 1.11 provides an overview of the methods involved in data reduction, followed by a business scenario illustrating how these methods can be applied in practice.
Method Name |
Description |
Feature selection techniques | Retains only the most informative variables by using methods such as correlation analysis (identifying and removing highly correlated variables) and chi-square tests (selecting features based on their statistical significance). |
Sampling | Extracts a subset of the data using techniques such as random sampling (selecting a random subset of data points) and stratified sampling (ensuring that the sample represents key characteristics of the population). |
Dimensionality reduction techniques | These techniques reduce the number of features through transformation or combination, such as Principal Component Analysis (PCA), which combines features into fewer components. |
Aggregation | Summarises data by grouping or combining records. |
Resampling for balancing skewed data | Adjusts the distribution of data classes using techniques such as oversampling (duplicating data points from underrepresented classes) and undersampling (removing data points from overrepresented classes). |
Clustering | Groups similar records together, allowing representative records (centroids) to stand in for the full dataset. |
Business scenario: Improving academic performance insights in the education sector
An education analytics company aims to analyse student performance data across various schools to identify factors influencing academic success. The data collected includes demographic information, grades, attendance records, extracurricular involvement and socioeconomic indicators. However, due to the large and complex nature of the dataset, the company applies several data preparation methods to streamline the analysis process.
- Feature selection techniques
The dataset contains variables such as “parental income”, “postcode, “number of siblings” and “study hours”. Using correlation analysis and chi-square tests, the company identifies that “study hours”, “attendance rate” and “parental education level” are the most informative features. Less relevant features, such as “postcode”, are excluded.
Result: By focusing on the most important variables, the analysis becomes more efficient and yields clearer insights into factors that impact student performance.
- Sampling
The company has data for 1 million students but wants to build a predictive model to identify students at risk of failing. To speed up processing, the company applies stratified sampling to ensure that the sample includes a balanced representation of high-performing, average and low-performing students.
Result: The smaller but representative sample allows for faster model development without compromising the quality of insights.
- Dimensionality reduction techniques
The dataset includes over 100 variables related to student activities, such as extracurricular participation, hours spent on homework and subject preferences. Using Principal Component Analysis (PCA), the company combines these variables into a smaller set of components, such as “academic engagement” and “extracurricular involvement”.
Result: The reduced dataset simplifies the analysis while retaining the key patterns and relationships needed to understand student success.
- Aggregation
The attendance records are collected daily for each student, creating a large volume of data. The company aggregates this data into monthly attendance rates for each student, summarising the information without losing its relevance.
Result: The aggregated dataset makes it easier to identify long-term attendance trends and their correlation with academic performance.
- Resampling for balancing skewed data
The company notices that the dataset is heavily skewed, with only 5 per cent of students categorised as “at risk of failing”. To balance the dataset, it uses oversampling to duplicate records of at-risk students and undersampling to reduce the number of high-performing student records.
Result: The balanced dataset prevents bias in the predictive model, ensuring that the system accurately identifies students at risk.
- Clustering
The company uses clustering techniques to group students based on their performance patterns, study habits and extracurricular participation. For example, one cluster might represent high-performing students with strong academic engagement, while another might represent struggling students with poor attendance.
Result: Clustering helps the company develop tailored intervention strategies, such as tutoring programs for struggling students or advanced coursework for high achievers.
1.6 Test your knowledge
References
- Ackoff, R. L. (1989). From data to wisdom. Journal of applied systems analysis, 16(1), 3-9.
- Microsoft. (2024). Copilot [Large language model]. https://copilot.microsoft.com/
Additional Readings for Australian case studies
- Agile Analytics. (n.d.). Tourism Australia Azure Power BI DAX solution case study. Retrieved January 24, 2025, from https://www.agile-analytics.com.au/stories/tourism-australia-azure-power-bi-dax-solution-case-study/
- BizData. (n.d.). Case studies. Retrieved January 24, 2025, from https://www.bizdata.com.au/case-studies
- CSIRO. (n.d.). Case studies. Retrieved January 24, 2025, from https://research.csiro.au/ads/case-studies/
- Dear Watson Consulting. (n.d.). Power BI case studies. Retrieved January 24, 2025, from https://www.dearwatson.net.au/power-bi-case-studies/
- DVT Group. (n.d.). Case studies. Retrieved January 24, 2025, from https://dvtgroup.com.au/case-studies/
- Expose Data. (n.d.). Case studies. Retrieved January 24, 2025, from https://exposedata.com.au/case-studies/
- NetSuite. (n.d.). Business intelligence examples. Retrieved January 24, 2025, from https://www.netsuite.com.au/portal/au/resource/articles/business-strategy/business-intelligence-examples.shtml
- SAP Concur. (n.d.). Case study. Retrieved January 24, 2025, from https://www.concur.com.au/casestudy
- Telstra Purple. (n.d.). Case studies. Retrieved January 24, 2025, from https://purple.telstra.com/insights/case-studies
- WingArc Australia. (n.d.). Case studies. Retrieved January 24, 2025, from https://wingarc.com.au/customers/case-studies/
Acknowledgements
Thank you to Khadeejah Atif who contributed to the design of images used in this chapter (Figure 1.1, Figure 1.2 and Figure 1.3)