4.9 Future directions
O’Reilly-Jacob et al. (2020) challenge us to consider that there is the potential for digital interventions to result in low-value care. The authors consider how a digital solution can provide suboptimal care if it is underdeveloped, when the solution supplements care rather than providing an alternative and when the solution provides unwanted care. As health service managers, we must consider how the digital solution will achieve genuine value gains for the consumer and the health and social care system.
In this section we will consider some of the future directions and challenges with a focus on:
- Emerging technologies in health and social care
- Anticipated challenges in the widespread adoption of technology
- Strategies for overcoming barriers and promoting continuous improvement
Emerging technologies in health and social care
Digital technology innovations offer the potential to solve current and emerging issues in the health and social care sector. Ensuring that digital solutions add value and solve problems is a key challenge for health service managers. The COVID-19 pandemic presented challenges and opportunities for digital health technologies. A range of technologies were rapidly deployed, such as mobile phone apps for contact tracing, data analytics, chatbots, symptom reporting apps, wearables and sensors, telehealth and drones (Budd et al., 2020).
Digital health technologies add value when they solve a problem and improve care and outcomes. Key to success is having the governance and management systems to ensure the technologies applied are safe and relevant and that the data generated is protected and useful.
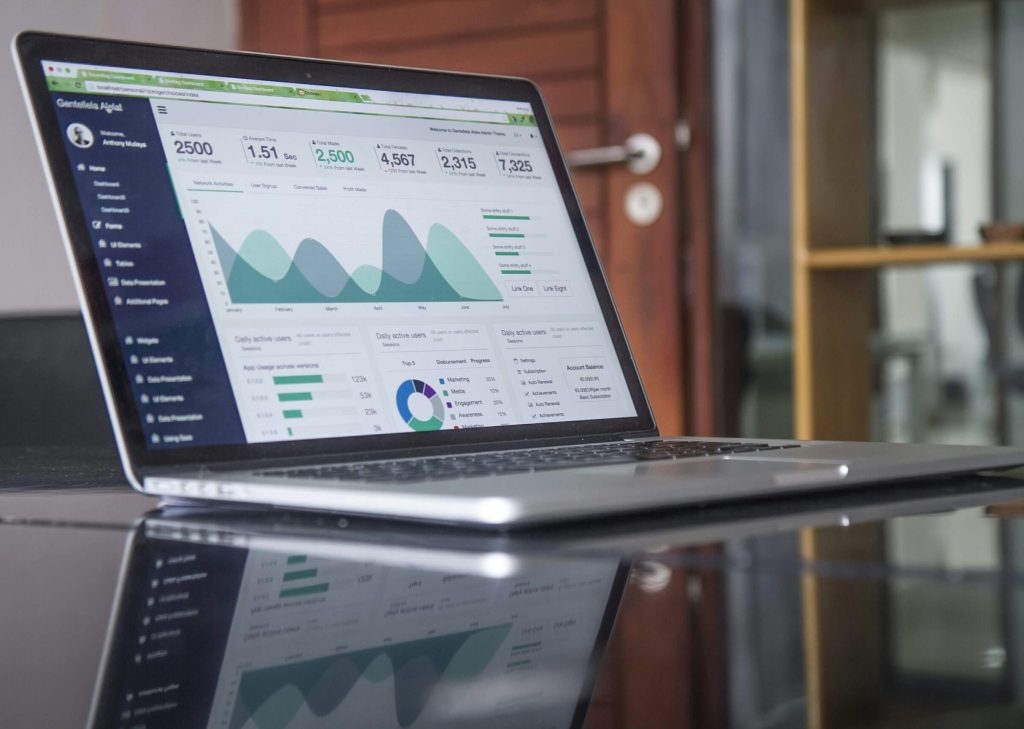
The OECD has reported that:
The development and proliferation of digital technology represents a seismic, worldwide transformation that is comparable to previous technological revolutions. The lifeblood of this digital transformation is data. Increasingly vast amounts of electronic data related to health and wellness are produced by healthcare systems, by government and private sector services, and by individuals though daily digital activities. These data – including what is referred to as Big Data – collectively hold much potential information that can foster improvement in all healthcare system activities, from clinical care to population health, to research and development in the life sciences industry. Taken together, more intelligent use of data can go some way to realising the ideal of the ‘learning health system’. (OECD, 2019, p. 214)
Many digital technologies offer benefits to the health and social care sector such as:
- Precision/personalised medicine / gene therapy
- Robotics: robotics aids in surgery by offering precision and minimally invasive procedures; in hospitals, robots help with cleaning tasks, ensuring thoroughness and reducing the risk of infection transmission
- 3D printing and miniature diagnostic devices: provide rapid, point-of-care testing capabilities, supporting early detection, efficient monitoring and timely intervention; non-invasive diagnostics such as swallowable cameras and targeted drug delivery can provide real-time monitoring and treatment options with minimal discomfort or invasiveness for patients
- MHealth, wearables; healthcare, rehabilitation and hospital in the home: receiving rehabilitation and healthcare at home can lead to significant cost savings by reducing hospital readmissions and minimising expenses associated with hospital stays; care in a familiar environment promotes comfort, independence and faster recovery
- AI and machine learning
We will now touch on a few of these.
Personalised/precision medicine
The British Heart Foundation (2022) defines personalised medicine as:
the process of tailoring medical decisions and interventions to an individual person. It’s about moving away from the one-size-fits-all approach and instead customising treatments for individuals.
VIDEO: PRECISION MEDICINE IN ACTION
Source: UC San Francisco (5 mins)
REFLECTION
Do you think health and medical research has made the progress expected in personalised medicine?
Where there has been progress, do you think that the health system is seeing the benefits and the value?
Personalised medicine can provide value for the healthcare system by averting late presentations for treatment and care, preventing adverse events, and through more targeted and responsive treatments. Examples include:
- shifting the emphasis in medicine from reaction to prevention
- predicting susceptibility to disease
- improved disease detection
- pre-empting disease progression
- customised disease-prevention strategies
- more effective prescription of medicines and drugs, including avoiding drugs with predictable side effects (Ahmed et al., 2020).
Love-Koh et al. (2018) found that three types of precision medicine are expected to emerge in clinical practice:
- Complex algorithms: the use of computer algorithms to support AI and clinical decision-making. These algorithms rely on large, complex datasets containing many variables (e.g. genetic data, sociodemographic data and the clinical detail in electronic health records).
- Digital health applications: primarily ‘app’-based solutions to support collection of patient-generated health data (e.g. physiological measurements).
- ‘Omics’-based tests: the use of genetic and genomic information about disease prognosis to predict treatment response. ‘Omics’ technology may become mainstream in healthcare (e.g. proteomics, metabolomics and lipidomics).
Precision medicine is already used:
- in cancer treatment (e.g. breast cancer, brain tumours, melanomas and leukemias)
- to treat known genetic disorders (e.g. forms of muscular dystrophy, Huntington’s disease)
- to treat autoimmune disorders (e.g. rheumatoid arthritis, Crohn’s disease).
Robotics
Robotics is the use of machines to perform tasks, including repetitive and complex tasks. It has been used in car manufacturing for many years; indeed many areas of manufacturing and logistics use robots. In healthcare, robotics is increasingly being applied to tasks that are repetitive or complex:
- Surgery – supporting precision and keyhole surgery
- Logistics – transporting medications, patient meals, surgical supplies
- Pathology laboratories – transporting specimens, processing large volumes of routine tests
- Treatment and rehabilitation therapies, including exoskeletons – supporting recovery from spinal and other traumatic injuries
- Patient monitoring, social and ‘care’ robots – supporting monitoring, social interactions and basic task completion
- Telerobotics supporting telehealth technologies
- Disinfecting devices and robotic cleaners
- Training clinical staff
Why is it important to consider how the field of robotics relates to value in digital health? From a health service management perspective, we need to consider the cost, the infrastructure required to support and integrate robotic technologies in a healthcare setting and the benefits that can be attained. For example, sensors and wireless transmissions to and from robots must not interfere with other equipment such as monitoring systems. Similarly, patient, resident and staff safety must be considered; autonomous mobile robots must not present a trip hazard. Wireless infrastructure includes robust wi-fi networks with sufficient coverage and bandwidth to support real-time communication. Secure and reliable communication protocols are essential to ensure the seamless operation of robotic systems while maintaining patient privacy and data security.
Cresswell et al. (2018) found that while there are significant opportunities for improving the safety, quality and efficiency of healthcare through robotics, there are also four major barriers that need to be effectively negotiated to realise these:
- No clear pull from professionals and patients
- Appearance of robots and associated expectations and concerns
- Disruption of the way work is organised and distributed
- New ethical and legal challenges requiring flexible liability and ethical frameworks
It is not just accommodating the hardware and software of the technologies; there is a socio-technological necessity to ensure that these technologies are integrated appropriately into the environment and the way care is provided.
3D printing
In healthcare, 3D printing has a range of current and emerging uses:
- Tissue/bio printing: skin, replacement organs and limbs.
- Anatomical models: complex anatomy 3D-printed in preparation for complex surgery so that surgeons can practice and refine techniques; to reproduce forensic anatomy; in neurosurgery 3D printing can be used to design customised implants or prosthetics for cranial reconstruction and fabricate surgical tools and guides for precise interventions.
- Pharmaceuticals: medicines delivered by nanotechnologies; custom-printed pharmaceutical delivery systems
- Medical devices and instruments: microneedles, replacement joints, biosensors
(Eshkalak et al., 2020)
Eshkalak et al. (2020) summarise some of the uses of 3D printing in health and medicine in Figure 4.5.
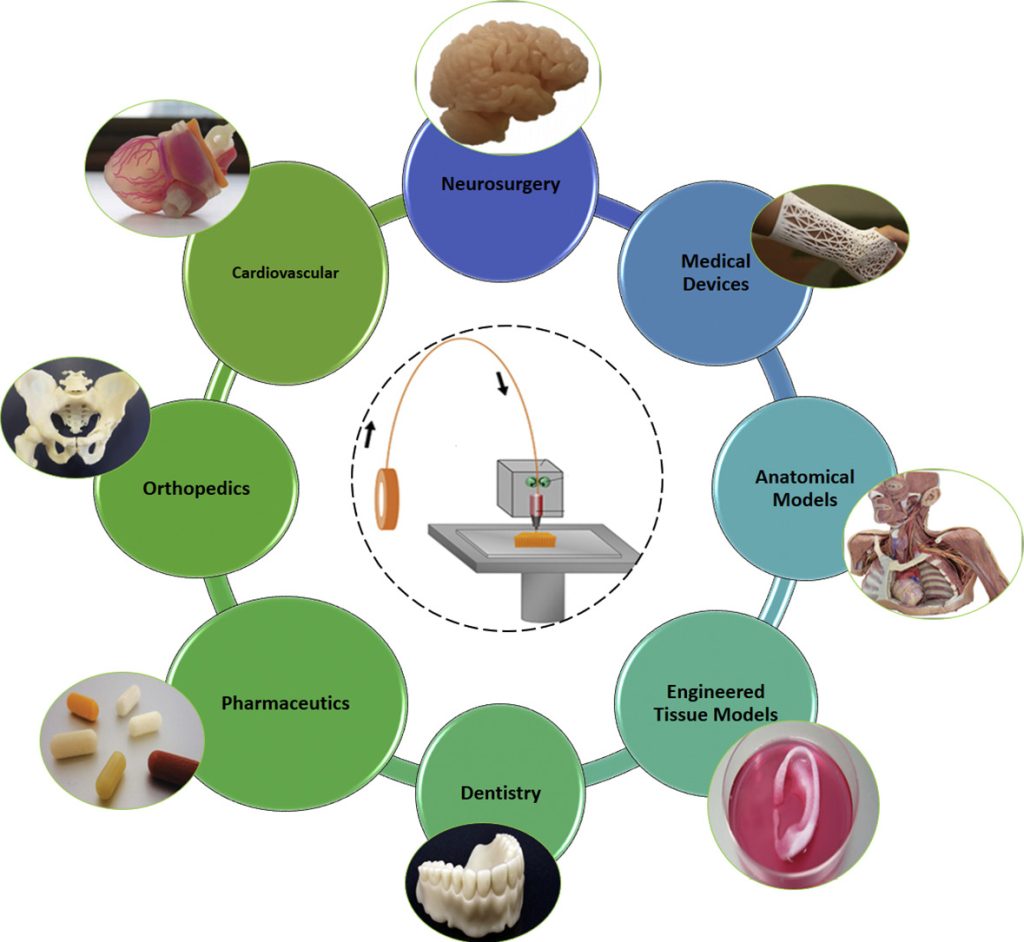
Source: Eshkalak et al. (2020). The role of three-dimensional printing in healthcare and medicine. Materials & Design, 194, 108940. Used under CC BY 4.0.
Eshkalak et al. (2020) explored the technical complexities for various approaches to 3D and future 4D printing, but at the centre of this innovation drive is the patient. Printing body tissues or organs relies on biometric data related to a specific patient. Similarly, printing complex surgical models (e.g. models of conjoined twins pre surgical separation) requires complex imaging . Such imaging consumes data storage and transmission capacity, making planning for long-term data management and storage a critical consideration (Eshkalak et al., 2020).
This field offers much in consumer and patient-centred care and treatments but must be governed by clear rules and solid research to ensure no harm (short or long term) is done.
Artificial intelligence
The concept of AI has been around since the mid-20th century when pioneers like Alan Turing and John McCarthy laid the theoretical groundwork. Since then, advancements in computing power, cloud computing, algorithms and data availability have propelled AI from theoretical concepts to practical applications. These include:
- reactive systems like IBM’s Deep Blue, which excels at playing chess
- limited memory systems such as autonomous vehicles, which use sensor data to navigate
- theory of mind AI, demonstrated by virtual assistants like Siri and Alexa, which understand and respond to human intent
- self-aware systems like Sophia the robot, capable of interacting with humans in a lifelike manner
- artificial narrow intelligence (ANI) like recommendation systems in online shopping platforms
- artificial general intelligence (AGI) systems, which aim to exhibit human-like intelligence across a wide range of tasks
- speculative artificial superintelligence (ASI), a theoretical concept representing intelligence surpassing human capabilities, yet to be realised.
(Joshi, 2019)
AI in health and social care presents many innovative opportunities, but with this technology come challenges and risks associated with ensuring genuine value-add in the sector. Challenges include long-term sustainability, including in infrastructures and infostructures, governance structures, and ethical and legal considerations.
FURTHER READING
If the potential for AI in healthcare is an area of interest, you may like to read this news release and a paper by Australia’s CSIRO.
CSIRO report highlights ‘extraordinary era’ of AI in healthcare
AI trends for healthcare report
CSIRO (March 2024)