5.2 Data characteristics
Data has been differentiated into several characteristics (Gantz & Reinsel, 2011). Many articles have proposed three to five characteristics (called also ‘the Vs’ of big data), although some literature cites up to 10 (Saeed & Husamaldin, 2021) (see Figure 5.1). The expansion in characterising data among data analytics researchers is mainly due to the breadth of available data and the continuous novel sources from which to retrieve it. The main purpose of health data is to provide timely, structured and accurate information for clinicians to support their decision-making (Saeed & Husamaldin, 2021).
The Vs of data that are often described in the literature refer to volume, velocity, value and variety of available data (Fan et al., 2013). These and six further Vs are outlined below (Ranjan, 2019; Saeed & Husamaldin, 2021).
- Volume: the volume of data available in each industry, sector or discipline. Data volume is growing considerably, and it is anticipated that the larger the data, the better the prediction models that will be created from them and the more reliable they will be.
- Velocity: the speed at which data is becoming available to provide real-time decision support information.
- Value: the ability to turn unstructured large data into usable, meaningful information relevant to the setting in which it is required.
- Variety: the type, diversity and source of data available. This involves data from structured and non-structured platforms in text, graph, audio and other forms.
- Veracity: refers often to the accuracy and timeliness of data available. Kepner et al. (2014) highlighted the importance of accurate data and having systems in place to ensure accuracies, since it is used, for example, as a base for important clinical decisions to manage patients in health settings.
- Validity: the correctness and relevance of the data for the purpose needed. It also refers to the rigour, credibility and quality of data available.
- Volatility: refers to the rapid and unpredictable change of data and, in some settings, to its replacement by more recent data. Some industries rely on constant refreshment, with recent data replacing older data. Commercial industries, the oil sector and share markets use volatility to make daily operational decisions.
- Variability: the correctness and accuracy of data obtained over time. It also refers to the unpredictability and lack of consistency of data. Systems managing data variability should be able to detect skewed data for reliability.
- Visualisation: the graphical representation of data in any form so it is easy to identify hidden patterns.
- Vulnerability: the security available for data to enable its protection and storage in accordance with relevant legislations and rules.
Some data characteristics are more relevant to specific industries than others (Anshari et al., 2019; Holmlund et al., 2020). Retail and oil and gas companies are the biggest users of most of the available data characteristics due to their dynamic environments and interdependencies on many external factors (Anshari et al., 2019, Holmlund et al., 2020).
On the other hand, in healthcare the data characteristics most frequently used are volume, velocity, variety, veracity and value (Dash et al., 2019; Saranya & Asha, 2021). When used in healthcare these characteristics can provide information that helps health services to develop, plan and implement interventions and evaluate their effectiveness (Borges do Nascimento et al., 2021; WHO, 2021). Using at least four characteristics (volume, variety, value and velocity) in healthcare provides an appreciation of the importance of the timeliness, complexity and size of available data to produce impactful change (Guo & Chen, 2023).
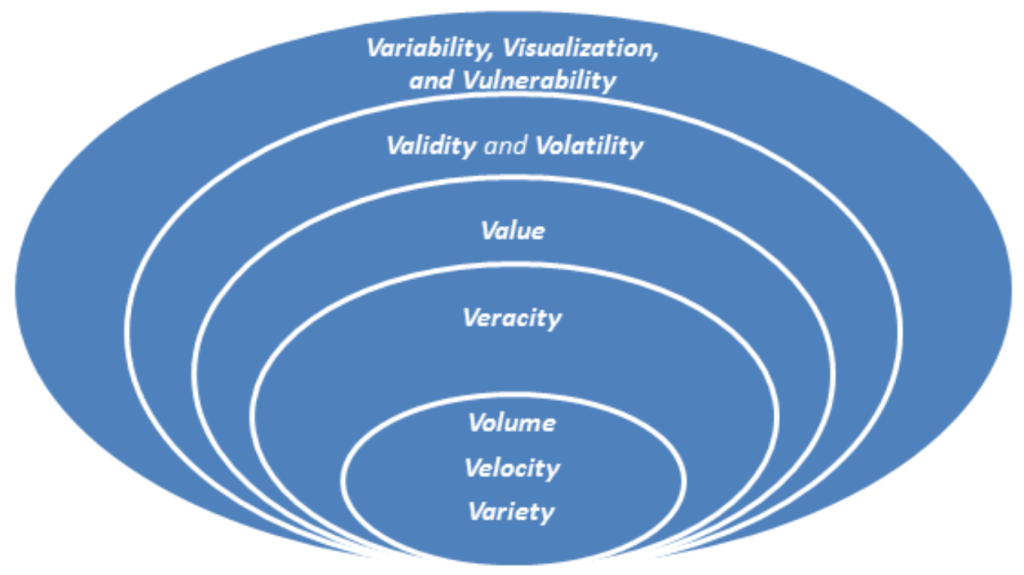
Source: Saeed, N., & Husamaldin, L. (2021). Big data characteristics (V’s) in industry. Iraqi Journal of Industrial Research, 8(1). Used under CC BY 4.0.
Analysing data is often done using a concept or model based on various assumptions and predictions that have been collected from previous research, experience or sometimes even from calculated or unexpected identification of relationships between various parameters of datasets (Watson, 2014). The science of data analytics has proven to be paramount in healthcare (Mehta et al., 2022). The recent COVID-19 pandemic has demonstrated the importance of timely data to healthcare providers to ensure appropriate clinical decisions are made in a dynamic environment to improve patient outcomes, where often patients’ lives are at high risk (Galetsi & Katsaliaki, 2020).