4.3 What can we do About it?
It is important to shed light on these questionable research practices to ensure that current and future researchers (such as yourself) understand the problems these research practices create for our discipline. However, in addition to highlighting what not to do, we should also talk about potential solutions to this so-called “crisis”. Easy changes we can make now include:
- Designing and conducting studies that have sufficient statistical power, in order to increase the reliability of findings.
- Publishing both null and significant findings (thereby counteracting the publication bias and reducing the file drawer problem).
- Describing one’s research designs in sufficient detail to enable other researchers to replicate a study using an identical or at least very similar procedure.
- Conducting high-quality replications and publishing these results (Brandt et al., 2014).[1]
Furthermore, there has been a movement to develop tools to help protect the reproducibility of scientific research. We will discuss each of them below.
Pre-Registration
One of the ideas that has gained the greatest traction is pre-registration, in which one submits a detailed description of a study (including all data analyses) to a trusted repository (such as the Open Science Framework or AsPredicted.org). By specifying one’s plans in detail prior to analysing the data, pre-registration provides greater faith that the analyses do not suffer from p-hacking or other questionable research practices. Pre-registration is a vital part of the Open Science Framework (see Figure 4.3.1 below).
The use of pre-registration in clinical trials has shown significant results. For example, the National Heart, Lung, and Blood Institute began requiring pre-registration of all clinical trials in 2000 through ClinicalTrials.gov. A study by Kaplan and Irvin (2015)[2] found that the number of positive results in clinical trials decreased after pre-registration was implemented, suggesting that pre-registration reduced the ability of researchers to manipulate their methods and hypotheses for a positive outcome.
Replication
As mentioned previously, the ability to replicate results is a critical aspect of science. To increase the likelihood of replicability, researchers should first attempt to replicate their own findings using a new, adequately powered sample. However, failure to replicate does not necessarily mean the original finding was incorrect. Multiple replications are needed to determine the validity of a finding. In the past, many fields, including psychology, have neglected this principle, resulting in “textbook” findings that may be false.
It’s important to note that p-values do not provide an estimate of the replicability of a finding. The p-value only reflects the likelihood of the data under a specific null hypothesis, not the probability that the finding is true. In order to know the likelihood of replication, we need to know the probability that the finding is true, which we generally don’t know.
Reproducible Practices
The paper by Simmons, Nelson, and Simonsohn (2011)[3] laid out a set of suggested practices for making research more reproducible, all of which should become standard for researchers:
- Authors must decide the rule for terminating data collection before data collection begins and report this rule in the article.
- Authors must collect at least 20 observations per parameter or else provide a compelling cost-of-data-collection justification.
- Authors must list all variables collected in a study.
- Authors must report all experimental conditions, including failed manipulations.
- If observations are eliminated, authors must also report what the statistical results are if those observations are included.
- If an analysis includes a covariate, authors must report the statistical results of the analysis without the covariate.
Doing Reproducible Data Analysis
The focus on replication so far has been on repeating experiments to verify findings by other researchers. However, computational reproducibility – the ability to reproduce someone’s data analysis – is also crucial. This requires researchers to share both their data and analysis code, allowing others to validate the results and test different methods. There is a growing trend in psychology towards open sharing and including the “open science badges” provided by the Centre for Open Science to encourage pre-registration and to share data, code and research materials.
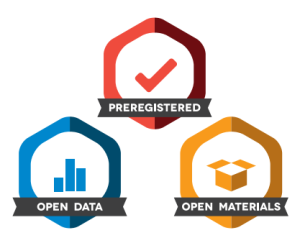
I also recommend using scripted analysis tools like R and free and open-source software (like jamovi!) rather than commercial ones, to promote reproducibility. Code can be shared on version control sites like Github, while datasets can be shared on portals like OSF.
Doing Better Science
It is every scientist’s responsibility to improve their research practices in order to increase the reproducibility of their research. It is essential to remember that the goal of research is not to find a significant result, rather, it is to ask and answer questions about nature in the most truthful way possible. Most of our hypotheses will be wrong, and we should be comfortable with that, so that when we find one that’s right, we will be even more confident in its truth.
Chapter attribution
This chapter contains taken and adapted material from Research methods in psychology by Rajiv S. Jhangiani, I-Chant A. Chiang, Carrie Cuttler and Dana C. Leighton, used under a CC BY-NC-SA 4.0 licence.
- Brandt, M. J., IJzerman, H., Dijksterhuis, A., Farach, F. J., Geller, J., Giner-Sorolla, R., Grange, J. A., Perugini, M., Spies, J. R., & van 't Veer, A. (2014). The replication recipe: What makes for a convincing replication? Journal of Experimental Social Psychology, 50, 217-224. http://doi.org/10.1016/j.jesp.2013.10.005 ↵
- Kaplan, R. M., & Irvin, V. L. (2015). Likelihood of null effects of large NHLBI clinical trials has increased over time. PloS one, 10(8), e0132382. https://doi.org/10.1371/journal.pone.0132382 ↵
- Simmons, J. P., Nelson, L. D., & Simonsohn, U. (2011). False-positive psychology: Undisclosed flexibility in data collection and analysis allows presenting anything as significant. Psychological Science 22(11): 1359–66. https://doi.org/10.1177/0956797611417632 ↵