3.3 What Makes a Good Measure?
It’s usually impossible to measure a construct without some error. For example, you may know the answer but misread the question and get it wrong. In other cases, the error is intrinsic to the thing being measured, such as in a simple reaction time test where the time it takes a person to respond can vary from trial to trial for various reasons. We generally strive to minimise measurement error as much as possible.
There are times when there’s a “gold standard” against which other measurements can be compared to. For example, sleep can be measured using various devices, such as those that measure movement in bed, but they are considered inferior to the gold standard of polysomnography, which uses brain waves to quantify the time a person spends in each stage of sleep. However, the gold standard is often more difficult or expensive to perform, so a cheaper method is used even though it may have greater error.
When evaluating the quality of a measurement, we generally distinguish between two different aspects: reliability and validity. Put simply, the reliability of a measure tells you how precisely you are measuring something, whereas the validity of a measure tells you how accurate the measure is.
Reliability
Reliability refers to the consistency of our measurements. One form of reliability is test-retest reliability, which measures how well the measurements agree if the same measurement is performed twice. For example, if a questionnaire is given to a person about their attitude towards statistics today, and the same questionnaire is repeated tomorrow, it would be expected that the answers would be similar, unless something significant changed the person’s view of statistics (like reading this book!).
Assessing test-retest reliability requires using the measure on a group of people at one time, using it again on the same group of people at a later time, and then looking at the test-retest correlation between the two sets of scores. This is typically done by graphing the data in a scatterplot and computing the correlation coefficient.
Another way to assess reliability is in cases where the data includes subjective judgments. For example, let’s say that a researcher wants to determine whether a treatment changes how well a child with Attention Deficit Hyperactivity Disorder (ADHD) interacts with other children, which is measured by having experts watch the child and rate their interactions with the other children. In this case, we would like to make sure that the answers don’t depend on the individual rater — that is, we would like for there to be high inter-rater reliability. This can be assessed by having more than one rater perform the rating, and then comparing their ratings to make sure that they agree well with one another.
Another kind of reliability is internal consistency, which is the consistency of people’s responses across the items on a multiple-item measure. In general, all the items on such measures are supposed to reflect the same underlying construct, so people’s scores on those items should be correlated with each other. On the dark triad personality test, if people’s responses to the different items are not correlated with each other, then it would no longer make sense to claim that they are all measuring the same underlying construct. This is as true for behavioural and physiological measures as for self-report measures.
Like test-retest reliability, internal consistency can only be assessed by collecting and analysing data. One approach is to look at a split-half correlation. This involves splitting the items into two sets, such as the first and second halves of the items or the even- and odd-numbered items. Then a score is computed for each set of items, and the relationship between the two sets of scores is examined. A split-half correlation of +.80 or greater is generally considered good internal consistency.
Perhaps the most common measure of internal consistency used by researchers in psychology is a statistic called Cronbach’s α (the Greek letter alpha). Conceptually, α is the mean of all possible split-half correlations for a set of items. Again, a value of +.80 or greater is generally taken to indicate good internal consistency.
Validity
Reliability is important but, on its own, it’s not enough. After all, I could create a perfectly reliable measurement on a personality test by re-coding every answer using the same number, regardless of how the person actually answers. We want our measurements to also be valid (see Figure 3.3.1) — that is, we want to make sure that we are actually measuring the construct that we think we are measuring. There are many different types of validity that are commonly discussed; we will focus on three of them.
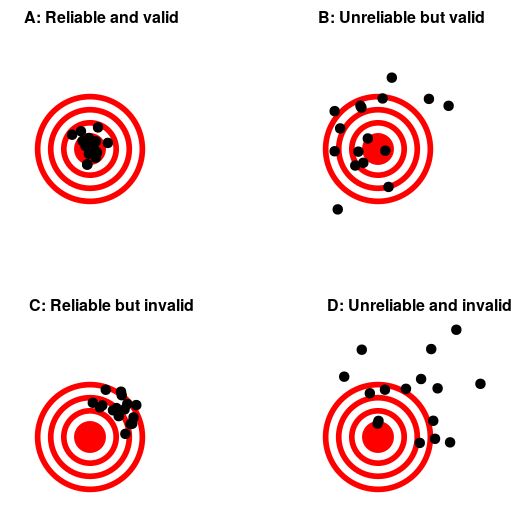
Face Validity
Does the measurement make sense at face value? If I were to tell you that I was going to measure a person’s blood pressure by looking at the colour of their tongue, you would probably think that, on the surface, this was not a valid measure. However, using a blood pressure cuff would have face validity. This is usually a first reality check before we dive into more complicated aspects of validity.
Content Validity
Content validity is the extent to which a measure “covers” the construct of interest. For example, if a researcher conceptually defines test anxiety as involving both sympathetic nervous system activation (leading to nervous feelings) and negative thoughts, then their measure of test anxiety should include items for both nervous feelings and negative thoughts.
Consider also that attitudes are usually defined as involving thoughts, feelings, and actions toward something. By this conceptual definition, a person has a positive attitude toward exercise to the extent that they think positive thoughts about exercising, feels good about exercising, and actually exercises. So to have good content validity, a measure of people’s attitudes toward exercise would have to reflect all three of these aspects. Like face validity, content validity is not usually assessed quantitatively. Instead, it is assessed by carefully checking the measurement method against the conceptual definition of the construct.
Construct Validity
Is the measurement related to other measurements in an appropriate way? This is often subdivided into two aspects: convergent and divergent validity. Convergent validity means that the measurement should be closely related to other measures that are thought to reflect the same construct. Let’s say that I am interested in measuring how extroverted a person is using a questionnaire or an interview. Convergent validity would be demonstrated if both of these different measurements are closely related to one another. However, measurements thought to reflect different constructs should be unrelated, known as divergent validity. If my theory of personality says that extraversion and conscientiousness are two distinct constructs, then I should also see that my measurements of extraversion are unrelated to measurements of conscientiousness.
Predictive Validity
If our measurements are truly valid, then they should also be predictive of other outcomes. For example, let’s say that we think that the psychological trait of sensation seeking (the desire for new experiences) is related to risk-taking in the real world. To assess the predictive validity of the sensation-seeking measurement, we would need to determine how effectively the scores on this test can predict scores on another survey that measures actual risk-taking behaviour (e.g., asking individuals if they would go sky-diving).
Assessing the Validity of a Study
When we read about psychology experiments with a critical view, one question to ask is, “is this study valid (accurate)?” Another one is to ask “can you trust the results of your study?” While the above types of validity are more applicable to measurements, we should also be assessing the validity of a study.
A study is said to be high in internal validity if the way it was conducted supports the conclusion that the predictor caused any observed differences in the outcome variable. Thus, experiments are high in internal validity because the way they are conducted — with the manipulation of the predictor and the control of extraneous variables (such as through the use of random assignment to minimise confounds) — provides strong support for causal conclusions. In contrast, non-experimental research designs (e.g., correlational designs), in which variables are measured but are not manipulated by an experimenter, are low in internal validity.
At the same time, the way that experiments are conducted sometimes leads to a different kind of criticism. Specifically, the need to manipulate the predictors and control extraneous variables means that experiments are often conducted under conditions that seem artificial (for instance, Bauman et al., 2014, had undergraduate students come to a laboratory on campus and complete a math test while wearing a swimsuit).[1] Furthermore, in many psychology experiments, the participants are all undergraduate students and come to a classroom or laboratory to fill out a series of paper-and-pencil questionnaires or to perform a carefully designed computerised task.
The issue we are confronting is that of external validity. External validity relates to the generalisability or applicability of your findings. That is, to what extent do you expect to see the same pattern of results in “real life” as you saw in your study? An empirical study is high in external validity if the way it was conducted supports generalising the results to people and situations beyond those actually studied. A very large proportion of studies in psychology will use undergraduate psychology students as the participants. Obviously, however, the researchers don’t care only about psychology students. They care about people in general. Given that, a study that uses only psychology students as participants always carries a risk of lacking external validity.
In saying that, however, a study that uses only psychology students does not necessarily have a problem with external validity (Navarro & Foxtrot, 2022).[2] Psychology undergraduates differ from the general population in many ways, therefore using only psychology students in a study may compromise its external validity. However, if the differences between the groups are not relevant to the phenomenon under investigation, then external validity should not be a concern. Navarro and Foxtroxt (2022) provided examples to make this distinction more concrete:
- You want to measure “attitudes of the general public towards psychotherapy”, but all of your participants are psychology students. This study would almost certainly have a problem with external validity.
- You want to measure the effectiveness of a visual illusion, and your participants are all psychology students. This study is unlikely to have a problem with external validity
Chapter attribution
This whole section contains taken and adapted material from several sources:
- Statistical thinking for the 21st Century by Russell A. Poldrack, used under a CC BY-NC 4.0 licence.
- Research methods in Psychology by Rajiv S. Jhangiani, I-Chant A. Chiang, Carrie Cuttler and Dana C. Leighton, used under a CC BY-NC-SA 4.0 licence.
- Fredrickson, B. L., Roberts, T.-A., Noll, S. M., Quinn, D. M., & Twenge, J. M. (1998). The swimsuit becomes you: Sex differences in self-objectification, restrained eating, and math performance. Journal of Personality and Social Psychology, 75, 269–284 ↵
- Navarro, D. J., & Foxcroft, D. R. (2022). Learning statistics with jamovi: A tutorial for psychology students and other beginners (Version 0.75). https://doi.org/10.24384/hgc3-7p15 ↵